In today's business world, the concept of growth is at the forefront of every organization's strategy. Companies are continually seeking innovative ways to expand their user base, revenue streams, and market influence. Central to this pursuit is the emergence of growth teams and growth products, whose primary focus is to drive and sustain the expansion of the business.
In this article, we will delve into the intricacies of creating effective growth teams and products, with a particular emphasis on the transformative role that AI products play in fueling this growth.
Gain a comprehensive understanding of how the synergy between growth teams and AI can be harnessed to propel businesses to new heights.
What is a growth PM?
There are several ways to define what a growth PM is, it's traditionally something that comes from a broader growth team. The focus here is really on driving value and optimizing flows and products that have not yet been optimized for growth.
So what does that mean?
If there's a certain value that a product is looking to drive for its members that can be measured in a metrics-driven way, then the problem that a growth PM needs to try and solve is - how do we get that value to the member more quickly?
The how is also something that is very unique to growth product management. The diagram above shows that there's actually a lot of different roles on a growth team. Traditionally, you have growth engineers, growth marketers, and specifically the growth PM is the one that works in the product management function on this team.
Growth metrics and ecosystem frameworks
There are some frameworks to cover here. Whenever we talk about growth, for a product or growth for a company, we have to talk about metrics, that's just necessary.
Traditionally, when you think of growth metrics, you think of this growth funnel, aka Pirate Metrics. Those are your acquisition metrics, activation metrics, retention, and then if applicable in a B2B type context, revenue and referrals.
So just to give some examples, acquisition is figuring out how to get people to start using your product. Some examples of this could be if you're using Uber, and you send another invite to a friend, to get them to start using this product. Activation is once they're in the product, how do you actually get them to start using it? Retention is once they've started using it, how do we keep the user onboard? Revenue is how do we generate revenue from those users, and referrals continue that cycle.
So that's traditionally how growth metrics have been defined. One of the newer ways is a loop model, which is less about driving people and users down into a certain funnel. It's more about how do we answer the question, how does your product grow? And so a lot of the times that question isn't answerable by just a strict funnel. It has to be more of a loop type model.
The image above is an example they give with Pinterest where you start with a new user, they get activated with some content, they pin some content, that content then gets indexed on search indexes like Google. Then your members actually find those pins by searching on Google without even necessarily looking for Pinterest in the first place. So it's more of a loop model in that context.
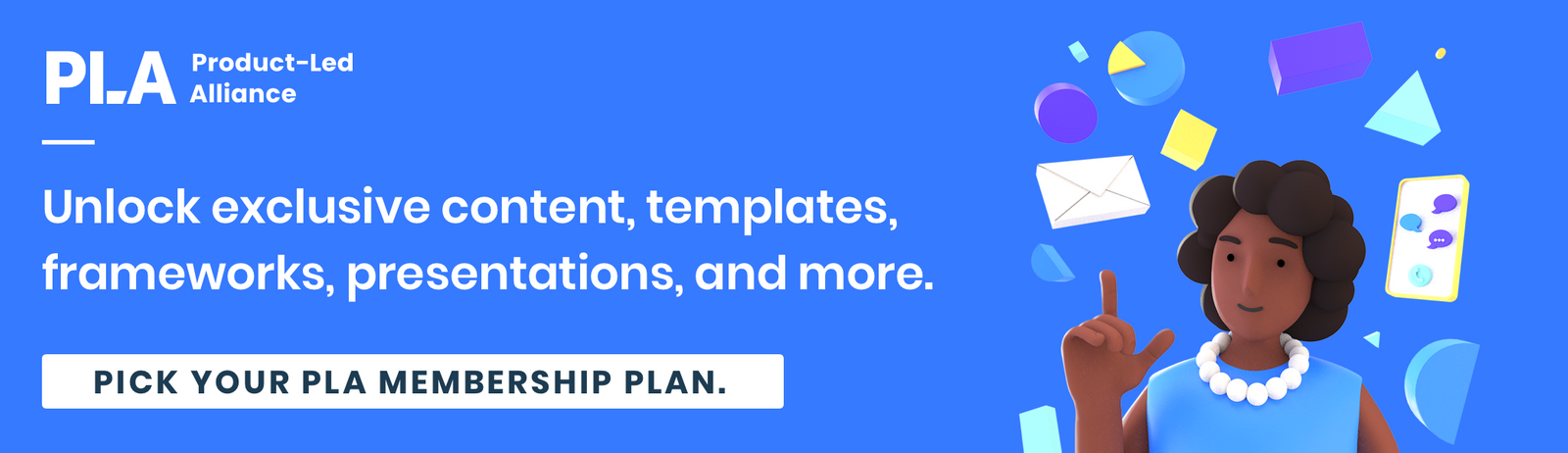
What makes a great growth PM?
Given that we have this definition for what a growth team is, and what a growth product manager does. Let's start thinking about what makes a good growth PM. The way that my team at LinkedIn likes to think about it, is there's three core categories to really define what makes a good growth product manager.
1) Ecosystem thinker
The first is that you are an ecosystem thinker. What this means is knowing why and where your team fits into the whole ecosystem. The reason why that's important is that as a product manager on a growth team, you're focused on driving value as effectively as possible. And you can only really do that if you understand where the levers are in the ecosystem.
In the image above is a diagram of the LinkedIn ecosystem where we have all our different products, hiring platform, our marketing platform, our learning platform. It's based on the foundation of having a professional network and a professional ecosystem of members who are all contributing and connecting to each other. This is really important, as a growth product manager when you start thinking about how to drive the value for the campaign manager, for example, and how to drive value for the hiring platform. It really helps to have an ecosystem mindset.
2) Data-driven decision maker
The second key factor in what makes a good growth PM, is you have to be data-driven. Data should backup all your decisions, all your hypotheses, you should never really be making decisions or having any hypotheses based on hunches. Being a good growth PM means being a data-driven decision-maker.
3) Experiment-driven learner
Finally, being a good growth PM means you're an experiment-driven learner, which means that you're acutely aware of what you want to learn. What is the problem that you're trying to solve? And what's the biggest assumption that you have around that problem? On the image above we also have a diagram of known unknowns.
If you look at the quadrant on the top right on the image above, you have known knowns, things that you definitely know. So for example, by reducing the onboarding flow by two steps, we can drive the retention rate by X per cent, which might be something that you already know, through an experiment. Then you have things that you don't know, but you know, you don't know them, and want to learn about them. The way that you solve for those and find out those learnings is through experimentation.
To summarise, what makes a good growth PM; being an ecosystem thinker, data-driven, and experiment-driven in order to learn things that you just don't know yet.
What is an ML/AI product?
Now that we've set some foundations on what we mean by growth product management, at least in this context, let's talk about let's shift gears and talk about what is a machine learning AI product, and then we can start talking about how they fit together.
The way that we define AI is human intelligence exhibited by machines. One of my favourite examples of how big this is, is that it changes a lot. In the past, when people were devising chess algorithms, they thought if a computer can beat a human at chess, that's human intelligence. Well, actually, no, that's still computer intelligence, we just got it wrong in the beginning.
Of course, around two years ago, Google's AlphaGo came out, beat the number one go player, and then they created another algorithm that could be that computer. The point is that the definition of AI is human intelligence exhibited by machines, but it's very much up to interpretation.
This leads to the second definition of machine learning, which is a lot more defined. Machine learning is actually the techniques and algorithms that use statistics to find patterns in large amounts of data. Generally, being able to form pattern recognition, and then predict things based on patterns is what humans do. So, if you can employ machine learning, you can generally do what a human can do, at least up to the level that we have now.
Then data science is a scientific method to extract knowledge and insights from data that can be fed into a machine learning algorithm. So these are just some definitions. Let's move on so that we can illustrate some of the other points on how it fits into product.
When to use ML in product?
Then the question becomes, when do we even use machine learning?
Machine learning and AI is a very hot topic that's thrown around a lot. Oftentimes it’s used in ways that it shouldn't be used. So let's focus on when you should use machine learning because it shouldn't be for everything.
“All you have is a hammer, everything looks like a nail.”
Basically, any problem that you're trying to solve is usually biased by the types of instruments that you have and the methods that you’re using to solve those problems. Let's dig a little deeper into what kind of problems are best suited for machine learning products.
What types of problems can ML help solve?
There are six types of machine learning problems here, they’re not exhaustive, and each of these can be broken out into other types of problems, too. But for the sake of this article, these are the six most common products and problems that you'll probably see in your day-to-day.
Let's talk about number one, ranking. What's the problem that we're trying to solve here? Usually, it's around helping users find the right thing. You can only really find the right thing if you've ranked the things first. So if you're looking for something on say Google search, you need to rank the things first, and then have a way to show the highest rank result in order to help a user find the right thing.
Another example might be feed ranking. LinkedIn’s feed is optimized to help members see the best posts based on the people that are connected to the things that they're connected to. And so the problem that they're trying to solve is providing the best quality post and most relevant posts through ranking.
The second machine learning algorithm has recommendations. The problem we're trying to solve here is how do we give users things that they may be interested in? Already, we started to see that there's never going to be a precise answer.
So in the image above, for example, we have our LinkedIn PYMK, which stands for people you may know. So it’s how do we show people that you may know so that you can connect to them?
For regression, the output of the regression algorithm is predicting an actual value of something for users. So one example could be a salary prediction. Let's say I'm job seeking, I'm going to be very interested in salary, and this is not this ambiguous value until a regression algorithm is used to provide that kind of prediction. It's a prediction based on other inputs and other data. So whether that be market information or previous historical data, the output is this actual value. That's what a regression algorithm does.
Our fourth is classification, helping users understand what kind of thing this is. So the example that we have here is being able to identify invitation spam. If you have a bunch of invitations being sent to you, a lot of them are not going to be relevant. How we define that is also something that needs to be done. Being able to classify what’s spam and what isn’t.
You also start to see that some of these overlap a little bit. So you might have a ranking algorithm that's based on recommendations. Next, we have clustering, which is based on putting similar things together for users to help them derive value, whatever that may be.
For example, on Amazon, “people also bought” is a grouping. The value that we're trying to drive there is people also bought this, so maybe this is something you're interested in purchasing. Another example is group two recommendations. On the PYMK front, it's like here are people you may know, based on a school that you've attended, or here are people that you know, based on your role, you’re a product manager here are the product managers. That's what clustering is.
Finally, we have anomaly detection, just finding uncommon things. A really interesting example is trending products. So if you look at Twitter, and people are talking more about Michael Jordan because there’s a documentary out, there's an anomaly. There's something strange because more people are talking about Michael Jordan than they normally would. Calling that out would be identifying that topic. These are the types of problems that machine learning can solve.
If you're thinking about when to use machine learning and product, it should really be around a certain problem that you're trying to solve. And generally, those problems you're trying to solve should fit into one of the above categories. There are other caveats, but generally speaking, that's how you formulate your problem.
ML product lifecycle - when to use, not to use
It's really important to think about the actual machine learning product lifecycle because understanding what is even required to develop a machine learning product should also drive your decisions.
The actual algorithm and product that you develop is highly dependent on the data that you have. Given that machine learning algorithms learn based on the inputs or data, you have to have the right data to begin with, in order to even begin developing a machine learning product.
So you formulate your problem, you select and pre-process the data that you have, which is a tremendous amount of data that you go through, so you have to label the data for it to be usable. If it's unstructured data, you have to be able to put it into a structured format that works for your team.
Step three is feature engineering, so given the problem and the objective function of the algorithm, how do we then decide what features to consider in the algorithm? For example, for people you may know, some features in the feature engineering set would be a school that you attended together, mutual connections, the industry that you're in. These are all part of the feature engineering process. Then for each of those, you have to decide how to weight them. The weighting should technically be done based on the data you're getting.
Then step number four is training, testing, and continuing to tune the models. So not only do you need this whole process, to begin with, once you've ramped the model. But as you continue to tune and update the models in real-time, you need to be super certain that that's something that your team can even support before pursuing a machine learning product.
When should you use a machine learning product?
When you're dealing with complex logic, that ideally cannot be resolved with if statements and business logic. Usually, that's because you need it to be personalized. So complex logic is necessary when you want your recommendations or your ranking to be personalized to each individual user. If you had to do that through business logic, that would be a nightmare to have enough cases and enough functions to deal with that.
Definitely use a machine learning product when you want it to be personalized. The third is probably one of the most key, which is that you want it to be in real-time. Your mutual connections change all the time, people go to different schools, they switch industries, change jobs. So if you want your product to be personalized in a real-time way, definitely use a machine learning product.
When shouldn’t you use a machine learning product?
As I mentioned in the whole ML product ML lifecycle, if you don't have enough data already, then you're going to have a number of issues. One is that you might just push through to create the model, but it's going to be a terrible model, and it's going to be extremely biased towards the small amount of low-quality data that you have.
There are tonnes of examples of products out there that used biased data and created a really poor experience for people. One example is the Microsoft Twitter bot that consumed a lot of vitriol and bad tweets and ended up becoming this very evil Twitter bot.
Definitely use machine learning if you want the solve very personalizable real-time problems. Don't use it if you can just use a hammer.
When can you use machine learning for growth?
Up to this point, we've discussed how growth product managers are looking to drive value as effectively as possible for the users, looking for optimization opportunities in the entire ecosystem of machine learning products, and are looking to solve complex personalized real-time problems.
A lot of interesting inventions or advancements in history have happened from combining two things that initially might have seemed different, into something that is completely new. A great example is the printing press. Gutenberg took a wine press, and the lettering system, which was manually putting together letters, and then combined them into this machine that could just press type very quickly. This led to the mass production of text, which led to the mass dissemination of texts, which led to knowledge.
So it's very cool whenever there are two different inventions that get combined into something that's very special. Personally, I feel that's where we're going when it comes to growth product management or just growth in general, and using machine learning products to drive growth.
Let's go back to the original criteria on what makes a good growth PM. So being an ecosystem, thinker, data-driven decision-maker, trend-driven learner - how do we use machine learning based on what we know so far to meet these criteria?
The ecosystem thinker
Asking yourself, how does machine learning play into this overall ecosystem? Where are all the levers? Where are all the inputs and outputs? And which of these places are ripe for using machine learning? A lot of time, you’ll find that it's perfect for machine learning.
Data-driven decision maker
Since data needs to backup all your decisions and hypotheses, where can we use machine learning to get as much data as possible and get all the right data we need to pick up decisions and drive hypotheses?
Experiment driven learner
Asking yourself, what do I want to learn? What are the biggest assumptions that I have and how can we use machine learning to find those insights that will then drive learnings.
Let’s go through the case studies that illustrate the above.
Case 1: people you may know, grouped reccs
Case one is people you may know, as grouped recommendations. So as I’ve mentioned, we have this product called people you may know, which is aimed at doing exactly what it suggests, showing you the people you may know.
One hypothesis that we had on the mind network team was that if we showed groupings of recommendations, those will be better recommendations and have more crisp insights into the people they may know. And that would lead to connecting more. So the hypothesis is very much around how we leverage this, our clustering, grouping, mixed with recommendations in order to provide the best recommendation.
As you can see in the image above, we've listed the hypothesis, we've also listed the ML that is leveraged in order to test this hypothesis. Then being very data-driven and experiment-focused in order to get those learnings is important as a growth PM.
So the metrics that we'd be measuring is member-to-member connections, that's our learning. Our experiment is if we do the groupings versus non-groupings, so the groupings would be one variant, and non-grouping would be the control.
In fact, within the groupings themselves, there are multiple variants. You can do groupings based on these different features, but you can also do groupings based on weight. Do we lessen the bar for what counts as a grouping? Do we be really strict about the quality? There are all sorts of different variations you could do within the model that lend themselves to driving these kinds of experiments.
The ecosystem thinking throughout all of this is that as we increase the quality of connections made, it's better for the overall LinkedIn ecosystem. You don't want an ecosystem of professionals based on very loose connections, you want it to be based on very strong connections, these clustered types of connections.
Going back to the ecosystem thinker, data-driven decision making, and experiment-driven learner. We can see that we really hit all those criteria with this experiment while leveraging machine learning.
Case 2: improving follows for engagement
The hypothesis here is that if we improve the recommendations that we show you for following, that will optimize the engagement. That in itself will lead to more engagement on the content that comes from those follows.
Take Steve Nouri in the recommendation examples in the image above, if I follow him, then I'll start seeing content from him, because he's a data science leader, and I'm very interested in data science. I'll see his content and my feed, and I’ll get notifications. I can then engage with that content, like and share, etc.
That is great for the ecosystem, as it’s encouraging a more active community on LinkedIn. But on the flip side, if I follow someone, and I see their content and it's not good or relevant at all, even though I did follow them, then that leaves a bad experience. Now I lose a little bit of trust in the recommendations being shown to me. As a member, I start questioning why these recommendations are being shown to me in the first place.
If we improve these recommendations, so that the ones being shown will optimize for engagement, then they'll lead to more content engagement. The machine learning algorithm here that we're utilizing is improving the existing recommendation model to do what we've listed above, which is optimizing for engagement. What that might mean, is making the prediction response of the recommendation algorithm optimized more for the actual engagement actions that a member has on the content from that individual recommendation.
Now we have members coming back to the platform. trusting the platform for quality content, which just leads to more community activity on the platform, which in turn helps out those other parts of LinkedIn, whether it be marketing, sales, etc. This is another case for how we might use machine learning in order to drive growth, and then help out the entire ecosystem.
Case 3: ranking recommendations
Case three is all about ranking the recommendations. On the my network page, you would see recommendations for different hashtag groups, people you may know, etc. But how do we actually rank those recommendations?
So our goal here is to provide the best recommendations at the top, similar to Google if you search for results. The goal and the problem we're trying to solve is making sure that the best recommendations are shown at the top of search results. Similarly, we want to show you the best recommendations and the best grouping of recommendations at the top.
Our hypothesis here is, if we rank recommendations based on the likelihood of the member engaging, we call that p(click) which is the probability that you'd click on those, then members will connect more and follow more things that they care about. This leads to a better ecosystem and so on.
The machine learning algorithm that we're leveraging here is a ranking algorithm. We are ranking based on the probability that you click on these things. This then helps out the overall ecosystem through more quality edges, we call an edge your connection to any of these things. So one edge might be following something, another edge might be connected to someone, another might be joining a group. So the more edges that we have, the more quality edges we have as well, which leads to a richer overall ecosystem on LinkedIn.
The metrics that we're measuring are what we call active edges; connections, follows, even subscriptions, all those are considered edges. So the more high-quality active edges that we can drive through this ranking experiment, the more that we would actually help out the overall ecosystem. This is a great example of how ranking is where growth and machine learning merge effectively.
In summary
ML/AI products are not one size fits all. The questions that you have to ask yourself here are what are the specific problems you're trying to solve in the first place. This is key because a lot of the time some are so well suited for machine learning, and some are just not suited at all. You need to ask yourself, does it need to be personalized, and does it have to be adaptable at scale? Does it need to be updating in real-time and learning on its own in order to solve the problem you’re solving?
In terms of combining growth and machine learning, it's really about asking yourself how you can improve the overall ecosystem using machine learning, and what you know about the types of problems that machine learning is best suited for. Look at the data you need to make product decisions, and how machine learning will get you that data.
Finally, look at your biggest assumptions and what kinds of ML model iteration experiments can help you get those learnings you need, which then in turn can help inform your future experiments. To make a better product and drive the most value for your users.
Hopefully, there's going to be a lot more exciting stuff to talk about and more new interesting case studies and examples. I'm really looking forward to that and I hope you are as well.
Thanks.
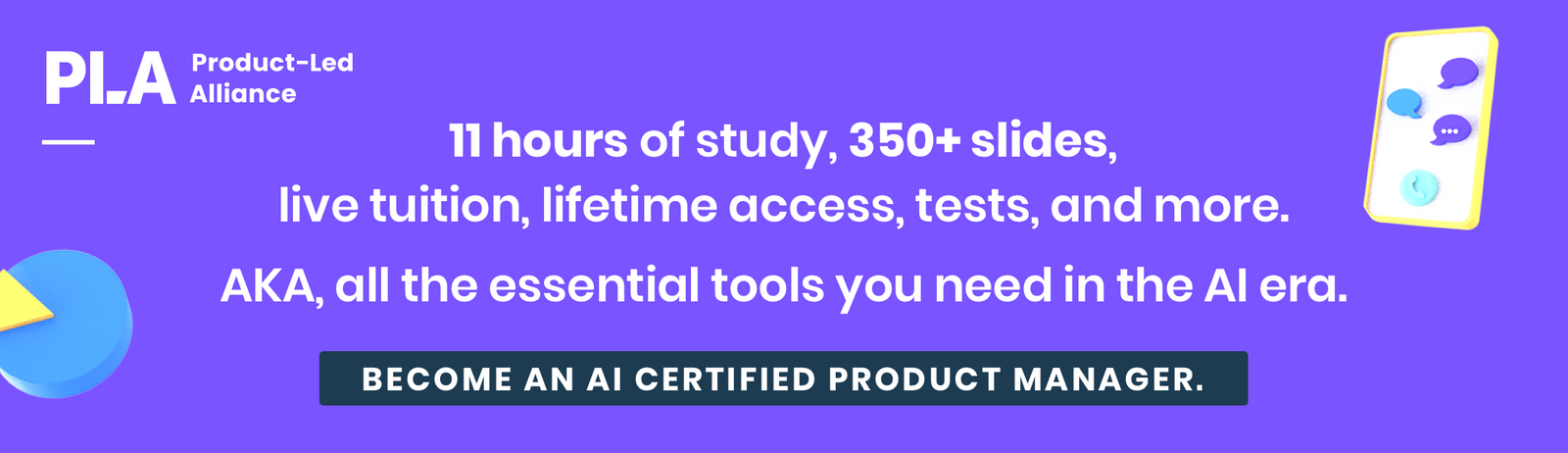