Artificial Intelligence (AI), once the domain of sci-fi novels and cutting-edge research labs, has rapidly transformed into a ubiquitous technology. Its evolution from an elite, specialized tool to a widely accessible commodity is reshaping industries, economies, and daily life in profound ways.
The journey of AI to commodity status
AI's journey to "commoditization" has been marked by several key developments. The last decade has witnessed exponential advancements in machine learning algorithms, computational power, and data storage capabilities. These technological leaps have made AI more efficient and scalable, driving down costs and broadening its accessibility.
The explosion of digital data from social media, IoT devices, and other sources has provided the raw material needed to train sophisticated AI models. This data abundance has enabled AI systems to achieve higher accuracy and effectiveness. The open-source movement has played a crucial role in democratizing AI.
Frameworks like TensorFlow, PyTorch, and Scikit-Learn, along with a wealth of pre-trained models, have lowered the barriers to entry, allowing developers and organizations of all sizes to harness AI capabilities.
The rise of cloud computing platforms like AWS, Google Cloud, and Microsoft Azure has made powerful AI tools and infrastructure available on-demand. This shift to AI-as-a-service means that even small businesses can leverage advanced AI without heavy upfront investments.
As AI continues to become a commodity, product managers face the challenge of prioritizing new technologies, products, and features to drive sustainable product-led growth. Here are some strategies and prioritization techniques to help manage this complex task effectively.
Prioritization techniques for AI and innovation
Value vs. Effort matrix
The Value vs. Effort matrix is a simple yet effective tool for prioritizing features and technologies based on their potential impact and the effort required to implement them.
- High Value, Low Effort (Quick wins): Prioritize these first. They provide the most significant return on investment and are relatively easy to implement.
- High Value, High Effort (Strategic initiatives): These are critical for long-term growth but require substantial resources. Plan and allocate resources accordingly.
- Low Value, Low Effort (Fill-ins): These can be tackled when resources are available but should not distract from more important tasks.
- Low Value, High Effort (Avoid): These should be deprioritized or reconsidered.
RICE scoring
RICE is a scoring model that considers Reach, Impact, Confidence, and Effort to help prioritize features.
- Reach: How many customers will be affected by the feature?
- Impact: How much will the feature improve the user experience or business metrics?
- Confidence: How confident are you in the estimates of Reach and Impact?
- Effort: How much effort in person-months will the feature take to implement?
RICE Score = (Reach × Impact × Confidence) / Effort
Features with the highest RICE scores should be prioritized.
Weighted scoring model
A weighted scoring model allows you to assign weights to different criteria based on their importance to your business goals. Criteria can include customer value, strategic alignment, revenue potential, competitive differentiation, and more.
- Assign weights to each criterion based on their importance.
- Score each feature against these criteria.
- Multiply the scores by the weights and sum them to get a total score for each feature.
MoSCoW method
The MoSCoW method categorizes features into Must-Haves, Should-Haves, Could-Haves, and Won’t-Haves.
- Must-haves: Essential features without which the product cannot function.
- Should-haves: Important features that are not critical but add significant value.
- Could-haves: Nice-to-have features that enhance the product but are not necessary.
- Won’t-haves: Features that are out of scope for the current cycle but may be revisited later.
Opportunity scoring (Outcome-driven innovation)
Opportunity scoring involves identifying underserved outcomes and scoring features based on how well they address these needs. This method helps focus on what matters most to customers and aligns product development with customer pain points and desires.
Strategies for sustainable product-led growth in AI and innovation
Customer-centric approach
- Continuously gather and analyze customer feedback to understand their needs and pain points.
- Use AI-driven analytics to gain deeper insights into customer behavior and preferences.
Agile development
- Adopt agile methodologies to quickly iterate on product features based on user feedback and changing market conditions.
- Implement AI tools to streamline development processes, automate testing, and improve efficiency.
Scalable infrastructure
- Invest in scalable cloud-based infrastructure to handle the increasing data and computational needs of AI applications.
- Ensure that your architecture can support rapid scaling as user demand grows.
Cross-functional collaboration
- Foster collaboration between product managers, data scientists, engineers, and other stakeholders to ensure alignment and leverage diverse expertise.
- Use AI collaboration tools to enhance communication and coordination among teams.
Continuous learning and experimentation
- Encourage a culture of experimentation and learning within your team. Use A/B testing and other methods to validate hypotheses and measure the impact of new features.
- Stay updated with the latest advancements in AI and related technologies to continuously innovate and improve your product.
Ethical AI practices
- Prioritize ethical considerations in AI development, including fairness, transparency, and privacy.
- Implement guidelines and best practices to ensure that AI systems are developed and used responsibly.
Focus on core competencies
- Identify and focus on your product’s core competencies and unique value propositions.
- Use AI to enhance these core areas rather than getting distracted by every new technology trend.
Conclusion
The commoditization of AI is still in its early stages, and its full potential is yet to be realized. As AI continues to evolve, it will likely become even more integrated into our daily lives, driving further advancements and efficiencies. Prioritizing new technologies, products, and features in the context of AI commoditization requires a balanced approach that considers value, effort, strategic alignment, and customer needs.
By employing techniques like the Value vs. Effort matrix, RICE scoring, weighted scoring models, MoSCoW method, and opportunity scoring, product managers can make informed decisions that drive sustainable growth.
Combining these techniques with a customer-centric approach, agile development, scalable infrastructure, cross-functional collaboration, continuous learning, and ethical AI practices will ensure long-term success and innovation in the rapidly evolving landscape of AI.
Are you ready to dive headfirst into the exciting realm where AI and product management collide?
Introducing our brand-new AI Product Manager's eBook – your ultimate guide to navigating the ever-evolving landscape of product management in the age of artificial intelligence.
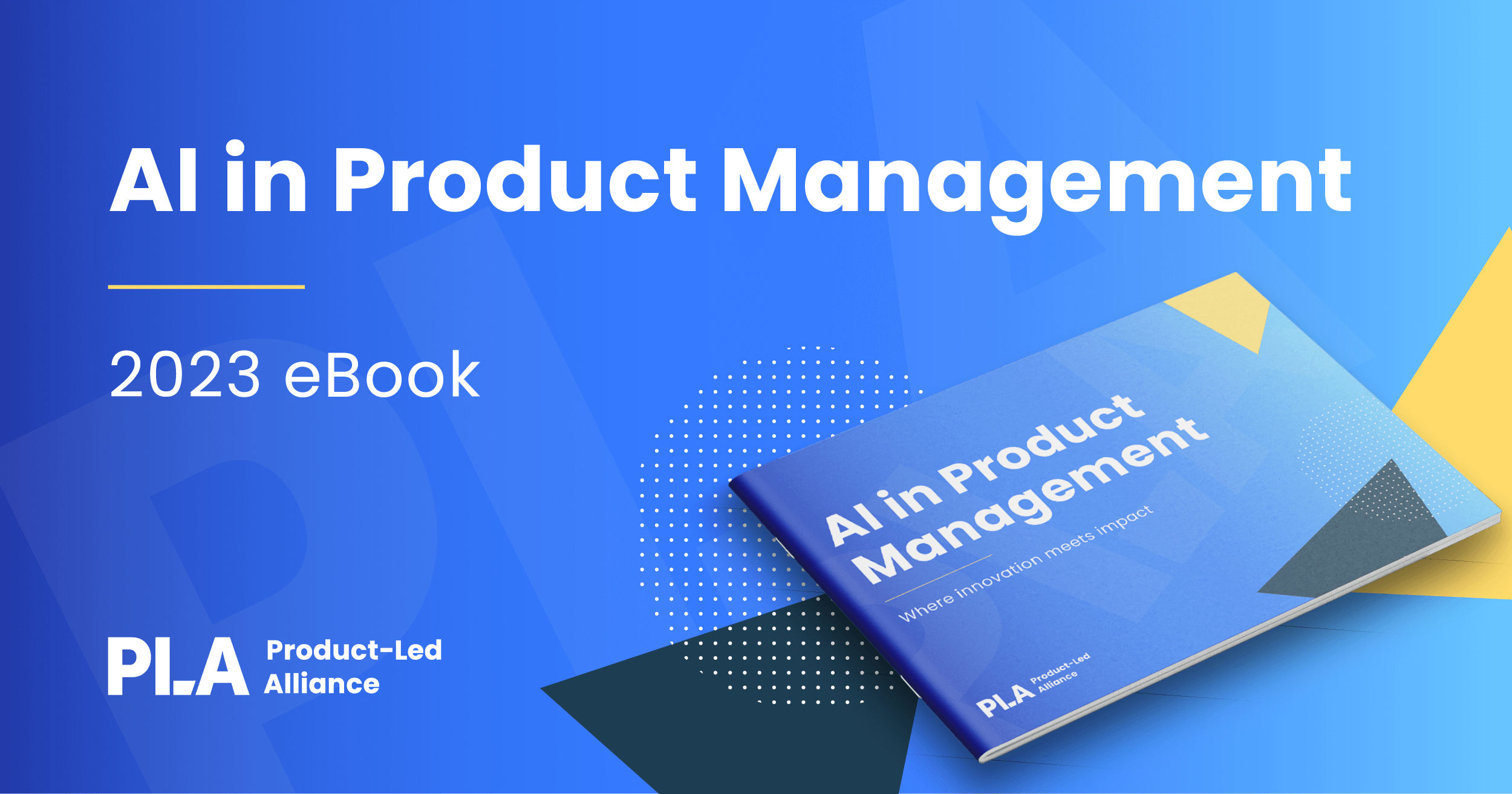