In recent years, artificial intelligence (AI) has undoubtedly become a game-changer, shaking up industries and sparking innovation worldwide. With AI constantly pushing boundaries, the role of AI product management has become increasingly important as product strategies adapt to the rate of technological change.
In fact, our latest AI product management report found that two-thirds of PMs are aiming to gain a competitive edge by implementing AI and machine learning functionality.
It's clear that AI product managers have a key role in connecting the dots between technology and business goals. They make sure AI-driven products not only meet customer needs but also bring value to the organization. In this guide, we'll dive into the nitty-gritty of AI product management, the challenges it poses, and strategies to thrive in this thrilling field.
Strategies for successful AI product management
The best AI managers are the ones with a holistic approach to the industry. They need to be well-rounded, not just from a technical standpoint but in their soft skills as well. Take a look and see if you’ve struck the right balance with your strategy, as laid out below.
1. Customer-centric approach: Understanding customer needs and pain points is essential for developing successful AI products. As an AI product manager, you should conduct thorough market research, gather user feedback, and continuously iterate based on customer insights to make sure your products deliver meaningful value.
2. Cross-functional collaboration: Effective AI product management relies on close collaboration with a diverse group of stakeholders, including data scientists, engineers, designers, and business leaders. By fostering strong communication and collaboration, you can effectively align technical capabilities with business objectives. This alignment is crucial for ensuring a smooth and efficient product development process.
3. Agile and iterative development: AI product management often involves dealing with complex and uncertain requirements, but don’t let that rock your boat. Adopting an agile development approach allows for quick iterations, frequent testing, and continuous improvement. This iterative process helps adapt to changing market dynamics, and user needs more effectively.
4. Lifelong learning: Given the rapidly evolving nature of AI, you need to have a growth mindset and a willingness to learn continuously. This involves staying updated on the latest AI advancements, attending industry conferences, participating in training programs, and networking with other AI professionals.
The challenges of AI product management
You understand what it takes to be an AI management pro, but what are the pitfalls that might creep up on you? By being aware of them, you can stay ahead of the game and circumnavigate the challenges when they arise.
In our latest State of AI Product Management report, we asked PMs to identify the key challenges involved in incorporating artificial intelligence into their workflows. 59% of respondents placed privacy concerns as the primary challenge, with over half stating that bias in data sets, data privacy, and transparency in decision should all be taken into account when using AI in product management.
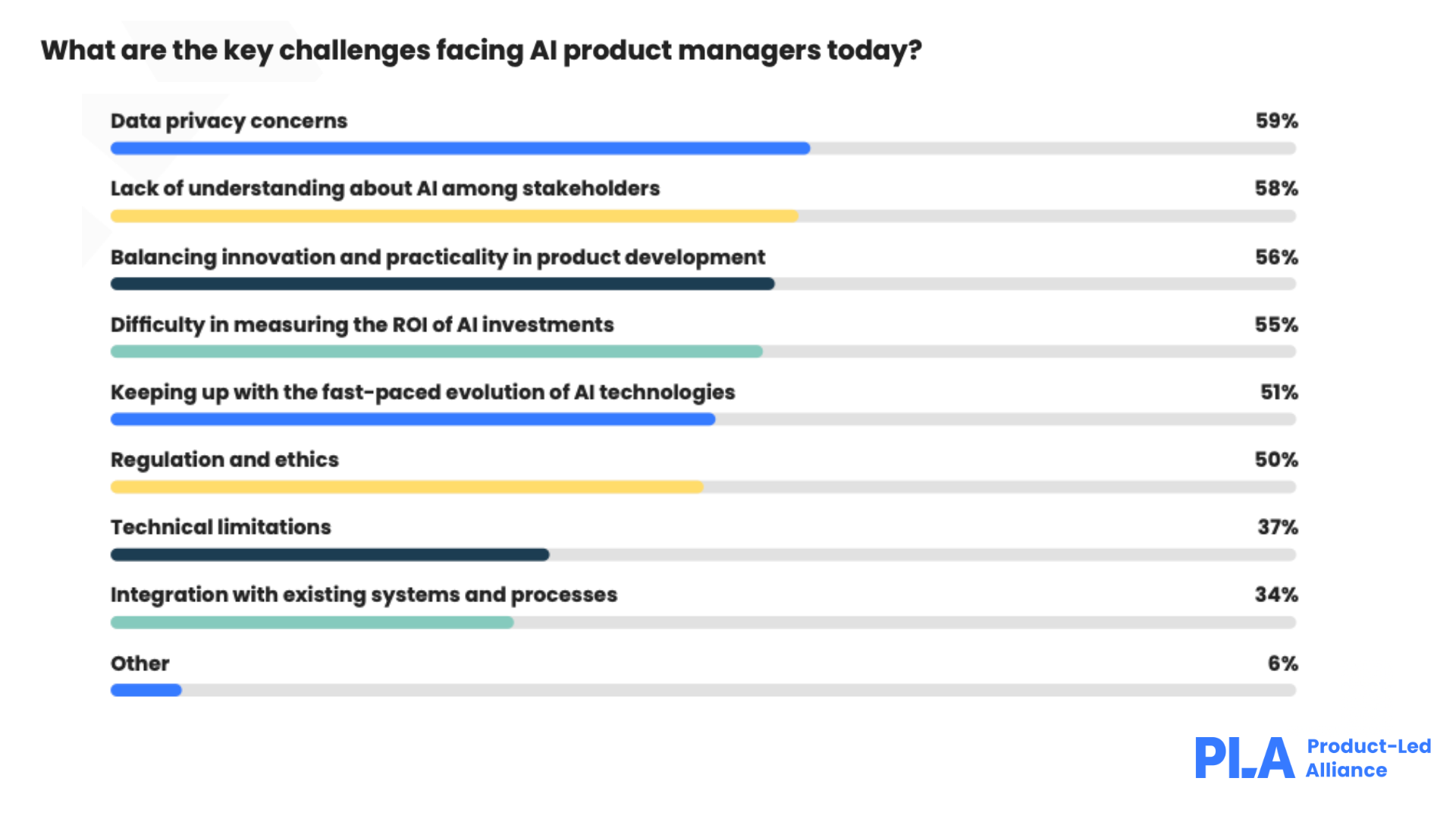
Based on our conversations with AI-focused product managers, here are the top challenges product teams ought to account for when leveraging the power of artificial intelligence.
1. Data quality and availability: AI relies heavily on high-quality data to train models and make accurate predictions. But obtaining clean, relevant, and diverse data can be a challenge, even for the best in the business. Good AI product managers work closely with data teams to ensure data quality, address biases, and establish robust data governance practices.
3. Uncertainty and complexity: AI technologies are rapidly evolving, and keeping up with the latest advancements can be daunting. AI product managers need to stay on top of industry trends, research breakthroughs, and changing customer expectations to make informed decisions and drive product innovation. You need to keep your finger on the pulse, so don’t become lax.
4. Regulatory compliance: AI products may be subject to regulations and compliance requirements, such as data protection laws and ethical guidelines. AI product managers need to work closely with their legal and compliance teams to ensure adherence to these regulations. You don’t want to end up in a mess you could have easily avoided with some due diligence.
Why do product managers need to embrace AI?
AI is taking over, though some companies are starting small while others are jumping in full course. Whether you’re ready for it or not, your company will probably be embracing the technology, so make sure you’re on top of it as you begin.
But why is it so important? AI is crucial for organizations to thrive in today's rapidly evolving business landscape. AI offers a myriad of benefits that can enhance the entire product lifecycle, from ideation to development, marketing, and customer support.
By leveraging AI-powered analytics and predictive modeling, you can gain valuable insights into market trends, consumer behavior, and competitive landscapes. This allows you to make data-driven decisions, identify untapped opportunities, and develop innovative products that cater to specific customer needs.
AI can also automate mundane tasks, such as data entry and analysis, allowing you to focus on strategic planning and creative problem-solving. And don’t forget, AI-powered chatbots and virtual assistants can improve customer engagement and provide real-time support, enhancing user experiences and fostering brand loyalty.
In today's fast-paced world, where speed, efficiency, and personalization are crucial, integrating AI into product management isn't just a choice but also a necessity for organizations aiming to remain competitive and provide outstanding products and services.
The role of an AI product manager
Nowadays, AI product management involves a multitude of tasks like data pipelining, exploratory analysis, MLOps, and more. It’s even brought along some fresh new roles, too, like data engineer, data analyst, and ML engineer. AI projects have become a real team sport, and the AI product manager is the ultimate head coach, calling the shots and making sure everything runs smoothly.
At the end of the day, the AI PM is still a product manager at heart, with a hint of AI expertise thrown in. The fundamentals of product management are still rock-solid and even more crucial in AI product teams. You gotta be a master of user knowledge and empathy, driving the execution of new systems and making data-driven decisions like a boss. These things are often overlooked, but they're the secret sauce in the world of AI product management.
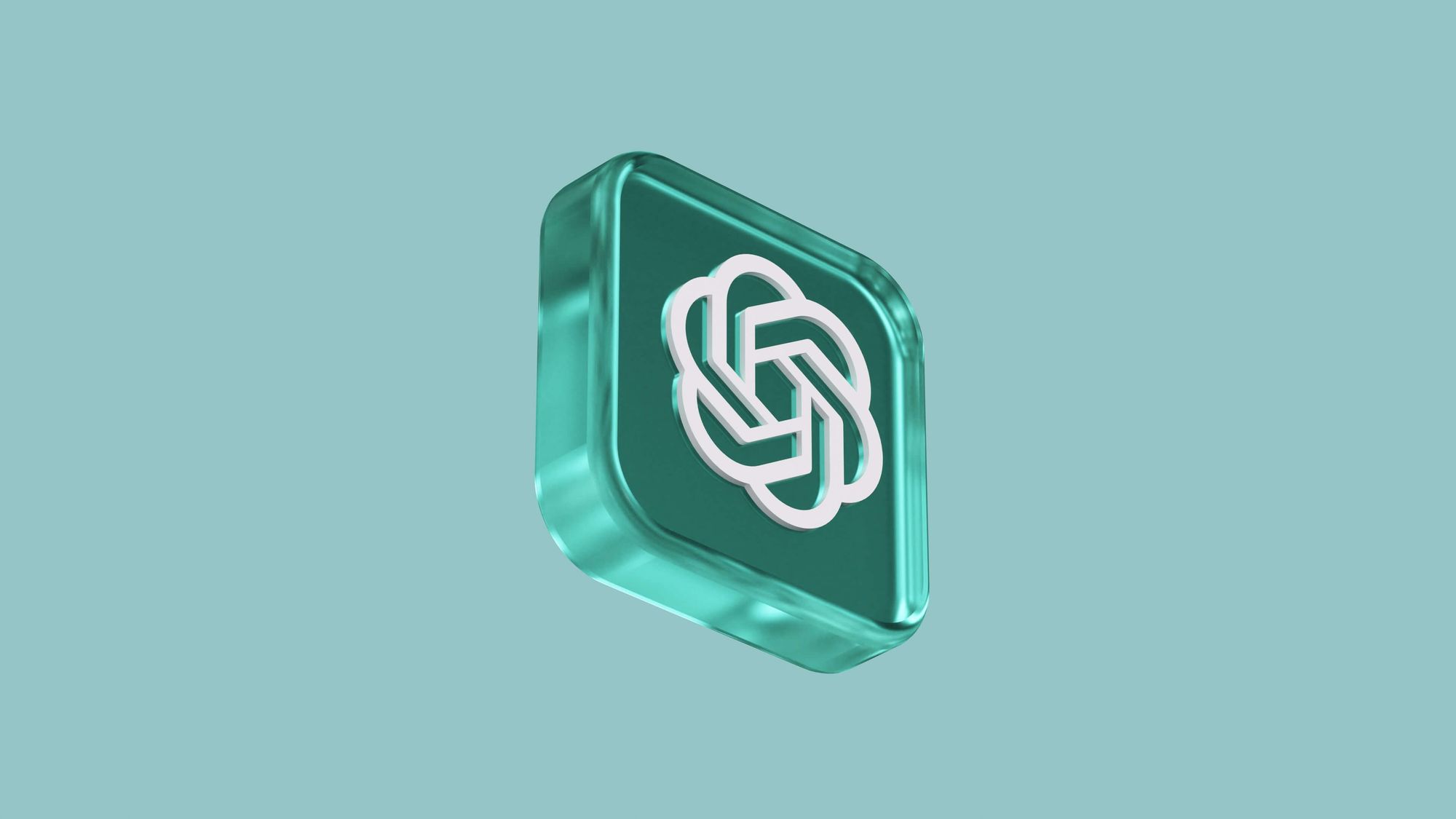
Marketing and product innovation
Let's dig into how AI can be the ultimate sidekick for PMs by making their lives a breeze throughout the product management journey.
Market and user research become simplified with AI-powered tools. They munch on massive amounts of data, spitting out insights on market trends and potential opportunities. And with Natural Language Processing (NLP) and sentiment analysis, they can dig into customers' minds, giving you a sneak peek into their opinions and preferences.
When it comes to idea management, AI systems are the ultimate organizers. They sort, categorize, and prioritize ideas based on set criteria. Plus, they're detectives, sniffing out patterns and relationships between different ideas and concepts. They'll have your ideas in shipshape order in no time.
Say goodbye to the hassle of creating technical specifications from scratch. AI swoops in and automates the generation of technical documentation, ensuring it's consistent and spot-on accurate. It's like having a tech-savvy assistant who never misses a beat.
Road mapping gets a turbo boost with AI-based algorithms. They analyze historical data, crunch numbers and predict the impact of specific features on user satisfaction, retention, and all those fancy KPIs. Let the algorithms do the heavy lifting, and you'll have the most optimized product roadmap in town.
Prioritization becomes a breeze with AI in your corner. It lends a helping hand in sorting out which features and tasks should take the front seat based on their potential impact on product success. Let the AI wizards do the math while you sit back and sip your coffee.
When it comes to product development, AI-based tools are the ultimate speed demons. They can generate code like nobody's business and catch those pesky bugs that hide in the depths of your software. With AI, development accelerates, and code quality skyrockets.
Minimum viable product (MVP) release and customer feedback collection become an adventure with AI. It takes user feedback, analyzes it like a champ, and unveils trends, spotlighting areas for improvement. And guess what? It even churns out fresh ideas for future iterations. Thanks to NLP and sentiment analysis, it goes beyond numbers and dives into the rich world of qualitative feedback.
So, buckle up and let AI be your trusty companion on this wild product management ride. It's here to make your life easier and sprinkle some magic on every stage of the process.
AI in product development
AI has truly transformed the product development landscape, enabling companies to create, refine, and enhance their offerings in remarkable ways. From the initial stages of ideation and design to prototyping and market testing, AI-driven product development streamlines processes, uncovers valuable insights, and delivers exceptional products that cater to ever-changing market demands.
Here are some key ways AI empowers product development:
1. Market research and customer insights: AI-powered tools analyze vast amounts of data from diverse sources like social media, customer reviews, and market trends. Techniques such as natural language processing and sentiment analysis provide valuable insights into customer preferences, opinions, and emerging market trends. This data-driven approach helps you better understand customer needs and design products that align with market demands.
2. Predictive analytics: AI algorithms excel at analyzing large datasets and identifying patterns. By leveraging historical data, AI algorithms can predict future trends, market demand, and customer behavior. This enables data-driven decision-making, such as selecting the most promising product features or identifying potential target markets, reducing risks and increasing the likelihood of success.
3. Personalization and recommendation systems: AI enables personalized user experiences by leveraging machine learning algorithms to analyze user behavior and preferences. This leads to personalized recommendations and suggestions, enhancing user engagement, customer satisfaction, and conversion rates. E-commerce platforms, for example, utilize AI-powered recommendation systems to suggest relevant products based on user browsing and purchase history.
4. Natural Language Processing: NLP allows products to understand and process human language, enabling features like voice assistants, chatbots, and sentiment analysis. Voice assistants like Siri and Alexa leverage NLP to interpret user commands and provide relevant responses. Chatbots engage in natural language conversations with users, offering customer support or assisting in the product selection process. Sentiment analysis helps gain insights into user sentiment by analyzing customer feedback, social media posts, and reviews.
5. Automated testing and quality assurance: AI automates testing processes, reducing the time and effort required for manual testing. Machine learning algorithms learn from historical test cases to automatically generate test scripts, speeding up the testing process. AI-powered tools analyze code data to identify potential bugs and anomalies, improving code quality and reducing the risk of errors in the final product.
6. Virtual prototyping and simulations: AI enables the creation of virtual prototypes and simulations for testing and optimizing product designs and functionalities. Virtual prototyping saves time and resources by simulating real-world conditions and providing insights into product performance. AI algorithms analyze simulation data, optimize design parameters, and suggest improvements to enhance your product performance.
7. Workflow optimization: AI automates repetitive tasks, optimizes resource allocation, and enhances collaboration among team members. AI-powered project management platforms automate task assignment, schedule optimization, and resource allocation, improving workflow efficiency. AI algorithms analyze team performance data to provide insights for optimizing team dynamics, leading to better coordination and productivity.
By harnessing AI technologies in these various ways, product development teams gain a competitive edge, streamline processes, and deliver innovative, customer-centric products that meet the evolving needs of the market.
How to make AI work for you
Let’s cover a few steps on how to embrace AI and make its strengths work for you.
1. Smart feedback analysis: With AI, you can automatically analyze user feedback like reviews and comments. The clever algorithms can pick up patterns, sentiments, and common issues raised by users. This helps pinpoint usability problems and areas that need improvement, giving designers the knowledge they need to make better decisions.
2. Tracking user behavior: AI-powered tools can keep tabs on how users interact with your product. They monitor things like clicks, scrolls, and navigation patterns to identify bottlenecks and usability issues. This kind of data tells you how people are really using your product and helps you optimize its usability.
3. Automated testing: AI can even simulate user interactions and perform automated usability tests. Using machine learning algorithms, it can mimic user behavior, complete tasks, and give feedback based on predefined criteria. This saves you time and resources by allowing you to test different scenarios on a larger scale.
4. Voice and image recognition: Thanks to AI, voice commands and image recognition can be analyzed for usability. Voice recognition technology enables hands-free interactions, while image recognition algorithms can assess the effectiveness of visual elements. So, whether it's a voice assistant or an image-based navigation system, AI helps ensure a user-friendly interface.
5. Predictive testing: AI algorithms can learn from past usability data to predict potential issues and suggest improvements. By studying previous testing outcomes, AI systems can anticipate user behavior, identify common pitfalls, and provide recommendations to enhance usability. This way, designers can make informed decisions and address potential problems before they even happen.
By leveraging AI in usability testing, you can streamline the process, gain valuable insights, and create products that are highly usable and loved by your users. It's all about making the testing experience smarter and more user-friendly.
What are other unique ways AI can help you?
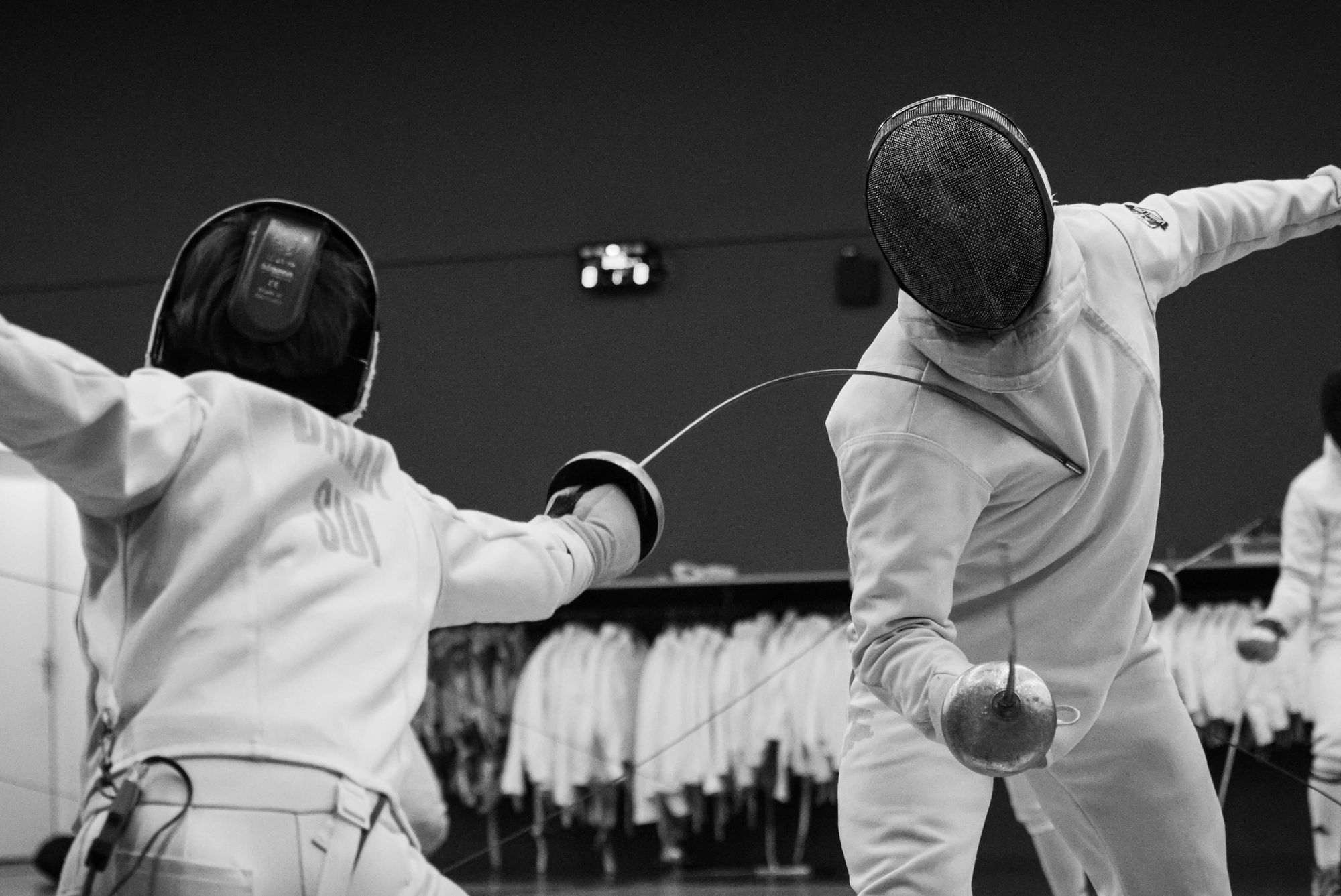
AI prompts for product development and decision-making
Let's explore some AI prompts that can empower you to excel in your role.
1. Market research and analysis
AI prompts can significantly streamline the market research and analysis process like it's magic. You can use AI to gather valuable insights about market trends, customer preferences, and competitor activities. For instance:
- "What are the current trends in [industry/market]?"
- "Identify key pain points of [target audience]."
- "Analyze competitor strengths and weaknesses in [product domain]."
By using AI to obtain real-time data and comprehensive reports, you can make those tricky decisions based on a thorough understanding of the market landscape.
2. Idea generation and validation
AI prompts can help you generate and validate new product ideas easily. By feeding AI with specific parameters, you can receive innovative concepts tailored to your target market and business goals. Some prompts may include:
- "Suggest product ideas for [target audience] that solve [specific problem]."
- "Evaluate the viability of [proposed feature/innovation] based on market demand."
- "Assess potential risks and benefits of implementing [new idea]."
With AI-generated ideas, you can quickly explore a wide range of possibilities and focus your efforts on concepts with the highest potential possible.
3. Customer insights and feedback
Understanding customer feedback is crucial for product improvement. You don't want to miss out on this one. AI prompts can assist you in analyzing customer sentiments and feedback from various sources, such as social media, surveys, and product reviews. Some prompts might include:
- "Analyze customer sentiment regarding [product/service] in [specific region/market]."
- "Identify common customer complaints related to [product feature]."
- "Summarize customer feedback on [recent product update]."
By leveraging AI to process and interpret vast amounts of customer data, you can identify areas for improvement and prioritize features that resonate most with your user base.
4. Predictive analytics for demand forecasting
With prompts, AI can provide predictive analytics for demand forecasting, enabling you to anticipate future market demand and plan inventory accordingly. It's like having a crystal ball. Some relevant prompts include:
- "Predict sales volumes for [product] in the next [time period]."
- "Forecast demand fluctuations based on historical sales data and external factors like seasonality or economic trends."
With AI-generated demand forecasts, you can optimize production and supply chain management, reducing the risk of overstocking or stockouts.
5. Competitive analysis and pricing strategies
Competitive analysis is essential to position your products effectively in the ever-changing market. AI prompts can help you assess competitor pricing strategies and devise optimal pricing models. For instance:
- "Analyze competitor pricing for [product category]."
- "Suggest competitive pricing strategies based on market dynamics and cost factors."
By using AI to gain insights into competitor actions and market conditions, you can set competitive prices and maximize profitability.
By leveraging the potential of AI prompts, you can elevate your impact on product development and create offerings that truly resonate with your target audience. Embracing AI is not about replacing human intuition but rather augmenting it with data-driven insights that can lead to better-informed decisions and more successful products.
Backlog grooming
Backlog grooming is like giving your product backlog a makeover in agile project management. It's when the team gets together to spruce things up and make sure everything's in good shape. You review the items on the backlog, break them down into smaller pieces if needed, toss out any outdated stuff, and put things in the right order of priority. It's all about keeping things organized and ready for action, so when it's time to plan the next sprint, you’re all set and good to go.
AI can be used to assist with backlog grooming by automating certain tasks, providing insights, and improving the overall efficiency of the process. Here are a few ways AI can be utilized for it:
1. Prioritization: AI algorithms can analyze various factors, such as user feedback, business goals, historical data, and market trends, to help you prioritize backlog items. By considering these factors, AI can provide recommendations on which items you should give higher priority, ensuring that the most valuable items are addressed first.
2. Natural language processing: You can use NLP techniques to analyze user stories, bug reports, and other backlog items. AI-powered NLP models can extract key information, identify dependencies, and categorize items based on their characteristics. This can help you in organizing and grouping similar items together, making the process more efficient.
3. Automated data entry: AI can assist with your backlog items by automatically extracting relevant information from various sources like emails, customer feedback, and support tickets. It can parse this information and populate the backlog with the necessary details, saving you time and reducing the chances of pesky human error.
4. Predictive analytics: AI can leverage historical data and patterns to predict potential risks, estimate effort, and forecast delivery timelines for your backlog items. These predictions can help you in making informed decisions about prioritization and resource allocation.
5. Intelligent recommendations: Based on the backlog items, AI can provide you with recommendations for potential solutions, alternative approaches, or suggested dependencies. It can analyze patterns from past successful implementations you’ve achieved and suggest similar solutions for new backlog items.
6. Collaboration and feedback: AI-powered collaboration tools can facilitate communication and feedback during backlog grooming. These tools can provide you with suggestions, navigate discussions, and capture feedback from your team members, helping to ensure that the backlog items are thoroughly reviewed and refined.
It's important to note that while AI can provide valuable support, your involvement and decision-making remain crucial in backlogging. AI should be seen as an augmentation tool, assisting you in your work rather than replacing your expertise and judgment.
How AI can create videos for your product
In the fast-paced world of digital marketing, captivating visuals, and engaging content are essential for grabbing the attention of potential customers. Videos have become an incredibly popular and effective medium for product businesses to showcase their offerings.
But what if we told you that AI could now take the reins and create impressive videos for your products? AI has revolutionized the way businesses approach video creation, enabling you to produce compelling content quickly and efficiently.
Come delve into how AI is transforming the world of product marketing through video production.
1. Automate your video production: AI algorithms have revolutionized video creation by analyzing product images, descriptions, and relevant data to generate videos automatically. With computer vision and machine learning, AI systems understand the key features and benefits of your product, crafting visually stunning video clips. Say goodbye to labor-intensive editing and expensive production costs as automation streamlines the entire process.
2. Personalize and target your videos: AI-powered video creation allows you to tailor content based on individual user preferences and behaviors. By analyzing customer data and feedback, AI algorithms generate customized videos that resonate with each viewer. Whether it's showcasing specific product features, adapting video styles to match your target audience or personalizing product demonstrations, AI empowers businesses to create highly targeted and engaging videos, increasing the chances of converting viewers into customers.
3. Unleash your creativity with enhanced visual effects: AI takes your video creations beyond basic templates, unlocking your creativity. AI algorithms generate visual effects, animations, and seamless transitions that elevate the overall quality of your videos. From captivating motion graphics to smooth scene changes, AI adds a touch of professionalism and creativity to your product marketing videos.
4. Optimize in real-time: AI systems analyze user engagement data in real-time, providing valuable insights to optimize your videos on the fly. By monitoring viewer behavior, AI algorithms identify the most engaging video segments, understand what captures users' attention, and even suggest improvements for future iterations. This iterative feedback loop ensures you constantly refine and optimize your video content to maximize impact and effectiveness.
5. Connect globally with multilingual videos: AI-powered video creation easily adapts to multiple languages, opening doors to new markets and expanding your global reach. By utilizing AI's language translation capabilities, businesses can automatically generate video content in different languages, catering to diverse audiences worldwide. This global scalability allows you to connect with customers from various regions and cultures, strengthening your brand presence on a global scale.
By harnessing the power of AI algorithms, you can create visually stunning and engaging videos that captivate audiences, drive conversions, and amplify your overall marketing efforts. As AI continues to advance, we can expect even more innovative applications in video creation, revolutionizing product marketing and providing immersive and impactful customer experiences.
So, embrace the power of AI and unlock the potential of video marketing for your product business today!
In-app user behavior
Understanding how users behave within mobile apps is super important for optimizing products, enhancing user experiences, and driving engagement. And guess what? AI has taken the analysis of in-app user behavior to a whole new level.
Let's dive in and see how AI is revolutionizing this game.
1. Comprehensive data collection and processing: AI-powered analytics platforms are masters at collecting and processing boatloads of data on user behavior within mobile applications. They can capture and analyze everything from clicks and swipes to the time users spend on specific features. This gives you a full picture of what users are up to, helps uncover sneaky patterns, and points out areas that need improvement.
2. User segmentation and personalization: AI algorithms are like wizards when it comes to dividing users into segments based on their behavior and preferences. By analyzing how users interact and spotting patterns, AI can group them into different segments. This knowledge is gold because it lets you personalize your app's content, recommendations, and features to make each user segment feel special and engaged.
3. Predictive analytics and user insights: AI isn't just about looking at the past; it can also predict the future! With machine learning algorithms, businesses can anticipate what users will do, what they'll like, and even whether they're about to say goodbye. Armed with this crystal ball, you can be proactive, meet user needs, dish out personalized recommendations, and keep users happy and engaged for the long haul.
4. Anomaly detection and fraud prevention: AI algorithms are like watchdogs that can sniff out anomalies and catch potential fraudsters within your app. They're always on the lookout, monitoring user behavior and comparing it to what's considered normal. If anything fishy happens, like fake accounts, fraudulent transactions, or weird usage patterns, AI will raise the alarm. This keeps your app safe and users' data protected.
5. Real-time recommendations and notifications: AI-powered systems are the kings of real-time recommendations and notifications. They analyze user interactions and historical data to serve up relevant product suggestions, juicy content, or exciting features right when users are actively engaged with the app. This turbocharges user experiences, boosts engagement, and cranks up conversion rates by giving users personalized recommendations exactly when they need them.
AI is changing the game when it comes to analyzing in-app user behavior. It gives businesses powerful insights into what users are up to, what they want, and what makes them tick. So jump on the AI train, embrace data-driven decision-making, make your users happy, and leave your competition in the dust. It's time to unleash the full potential of analyzing in-app user behavior and take your mobile app success to the next level.
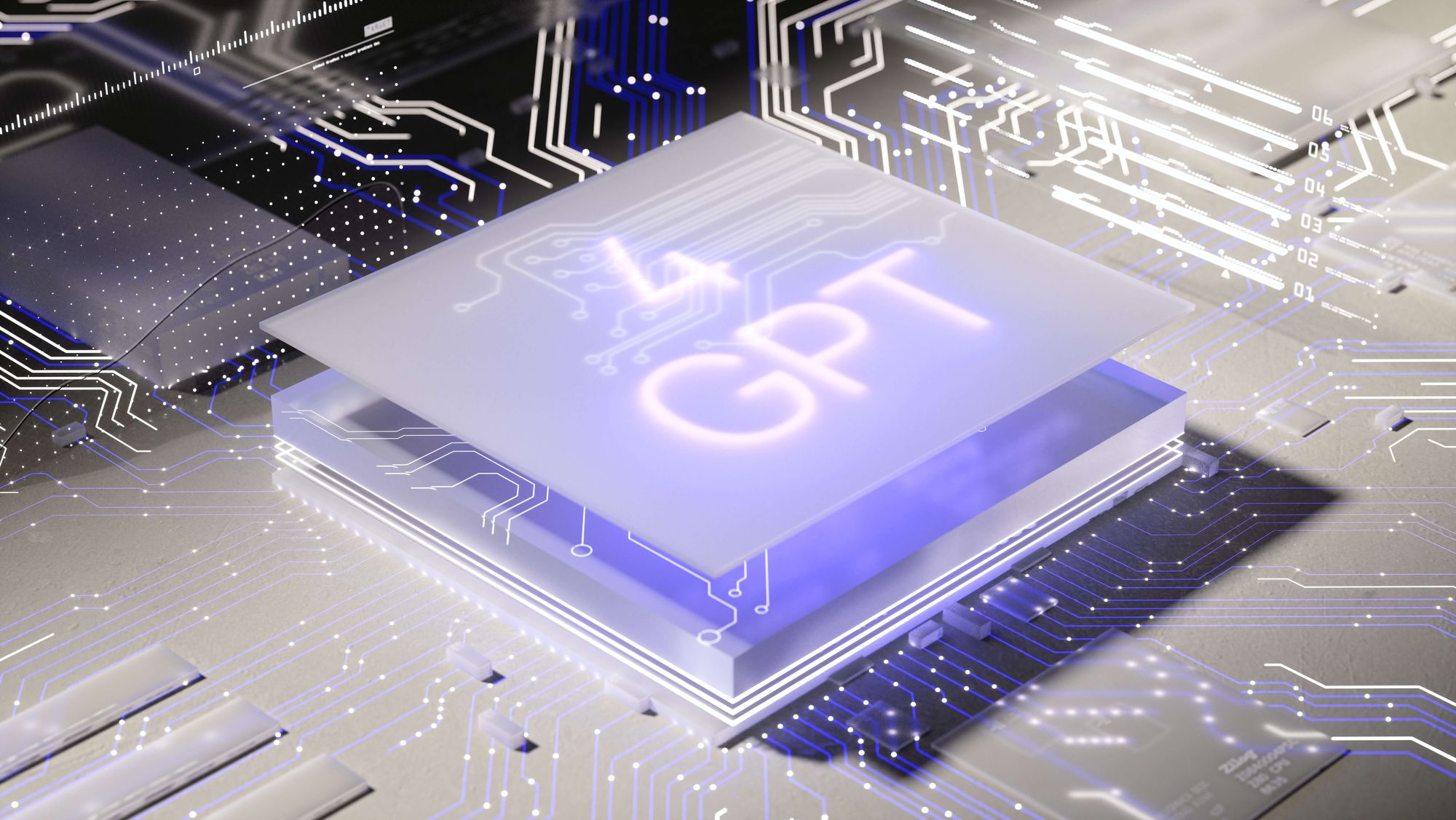
Feedback loops
AI plays a super important role in automating and closing feedback loops by making the process of collecting, analyzing, and acting upon user feedback way smoother. Let's check out how AI can automatically close feedback loops:
1. Automated feedback collection: AI-powered systems can scoop up feedback from all sorts of places like customer surveys, social media, support tickets, and online reviews. NLP algorithms can sift through the feedback and pull out valuable insights and sentiments, making it easier to organize and understand.
2. Sentiment analysis and topic extraction: AI algorithms can read between the lines of user feedback and figure out if it's positive, negative, or somewhere in between. They can also pick up on the main topics or issues users are talking about, giving businesses a clear idea of what's on their minds.
3. Prioritization and categorization: AI can sort feedback automatically based on stuff like product features, usability, performance, or customer service. This helps you spot recurring issues or things that need immediate attention, so you can set your priorities straight.
4. Automated routing and escalation: AI systems are smart enough to send feedback to the right teams or departments in your organization. So, if there's negative feedback about your customer service, it can be flagged and sent directly to your support team for a speedy resolution. This makes sure that the right people get the feedback and cuts down on response times.
5. Continuous monitoring and alerting: AI keeps an eye on user feedback in real-time, so you're always in the know about emerging trends or issues. It can spot any weird stuff going on, like sudden changes in sentiment or big spikes in specific topics, and give you a heads-up about things that need your attention ASAP.
6. Closed-loop communication: AI can help you communicate and follow up with users who give feedback. Automated systems can shoot off personalized responses, letting users know their input is appreciated and keeping them in the loop about what actions you're taking to address their concerns. This kind of communication shows users that you're serious about keeping them happy and helps build trust and loyalty.
By tapping into the power of AI in feedback analysis, you can close those feedback loops like a champ. User feedback gets collected, analyzed, and acted upon in a jiffy, allowing you to make your products, services, and overall customer experience even better. It's all about showing users you're listening and making their needs a top priority.
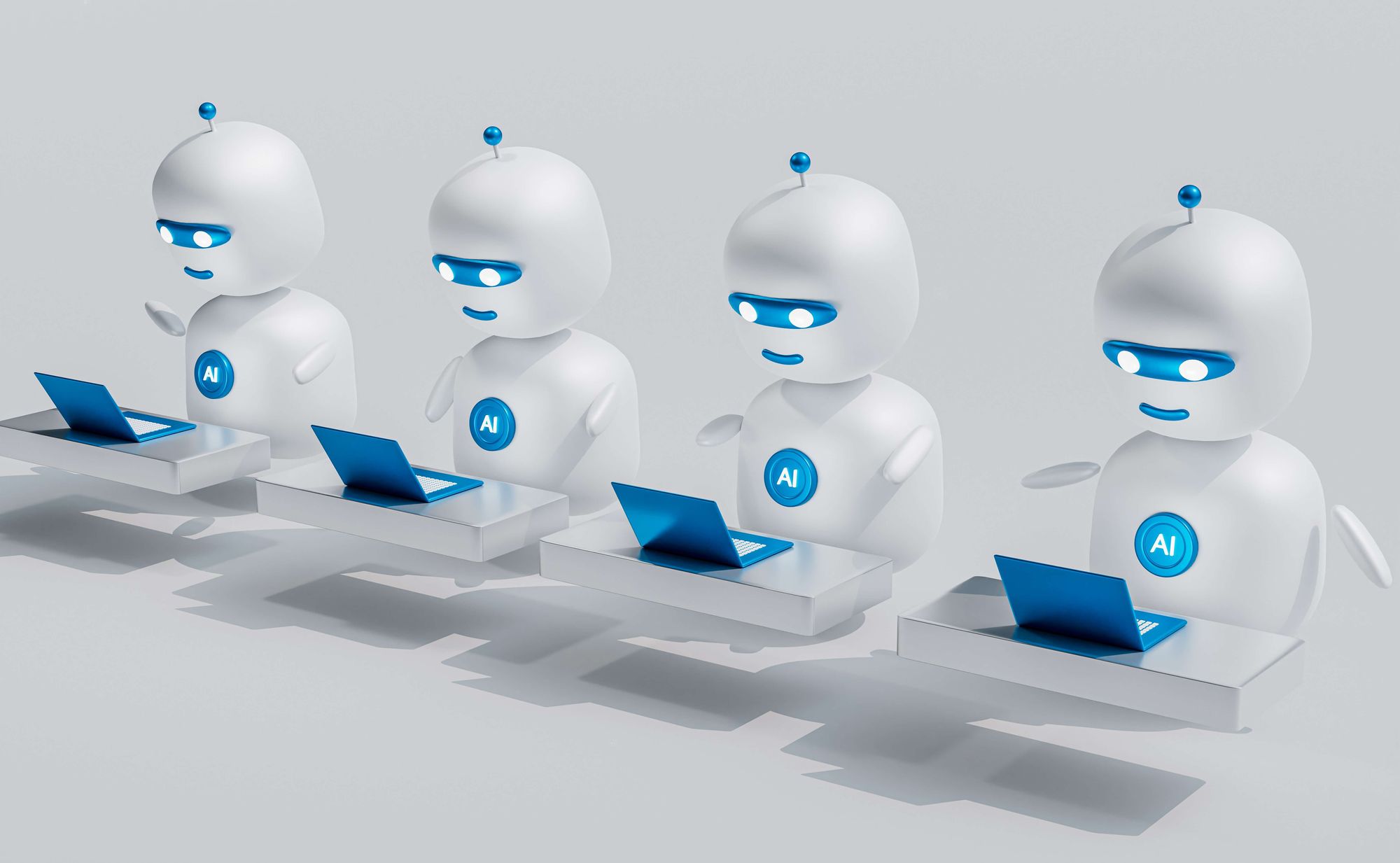
Unveiling the AI titans: comparing ChatGPT and Google BARD
When it comes to AI models, ChatGPT and Google BARD have emerged as major players, each bringing unique capabilities and applications to the table. So, let's compare these two AI giants and explore their features, strengths, and potential uses.
Understanding the AI models
Developed by OpenAI, ChatGPT is a seriously powerful language model that can whip up text responses that sound just like humans. It's built using deep learning techniques and has been trained on massive datasets, so it knows how to generate coherent and contextually relevant responses.
BARD (Bidirectional Encoder Representations from Transformers for Data-to-Text Generation) is Google's own AI model. It's all about converting structured data into good ol' natural language text. BARD uses the Transformer architecture to process and create detailed, well-written text based on the data it's given.
Language generation capabilities
ChatGPT is a master at generating conversational responses, making your interactions with it super engaging and dynamic. It's great at tasks like answering questions, explaining things, and coming up with creative text content.
Unlike ChatGPT, BARD is all about converting data into text. It's a pro at turning structured data into smooth narratives that flow naturally. It's perfect for generating reports, summarizing data, and creating personalized content.
Strengths and limitations
The real strength of ChatGPT lies in how well it can generate text that sounds human-like, giving you a seamless conversational experience. But sometimes it might not be totally accurate, or it might show some biases because of the limits of its training data.
BARD really shines when it comes to generating data-driven narratives. It's all about producing coherent texts based on structured inputs. However, it might struggle a bit when it comes to conversation or generating responses beyond the given data context.
Use cases and applications
You can put ChatGPT to work in virtual assistants, chatbots, content creation, and interactive storytelling. It's versatile and can handle a wide range of user-facing AI experiences.
BARD is the go-to for scenarios where you need to turn data into readable narratives. It's perfect for data reporting, business intelligence, personalized summaries, and transforming large datasets into text that's easy to understand.
Continued advancements and future directions
Both ChatGPT and Google BARD are constantly evolving through ongoing research and development. OpenAI and Google are putting in a lot of effort to address the limitations of their models, like refining biases, improving training data, hallucinations - where models offer inaccurate data - and expanding the range of potential use cases.
ChatGPT and Google BARD are seriously impressive advancements in AI technology, each excelling in its own language generation domain. ChatGPT rules in conversational applications, while Google BARD dominates in data-to-text generation scenarios.
By understanding their strengths and limitations, we can make the most of these AI models, opening up exciting possibilities in virtual assistants, content creation, data reporting, and beyond. As AI keeps evolving, we can expect even more sophisticated and versatile language models that will totally redefine human-computer interaction.
Conclusion
AI product management is a dynamic and challenging field that requires a unique blend of technical expertise, business acumen, and strategic thinking. As AI continues to reshape industries, organizations need skilled AI product managers who can effectively navigate the complexities and drive successful product outcomes.
AI product managers can maximize their chances of success in this exciting and rapidly evolving domain by adopting customer-centric approaches, fostering cross-functional collaboration, embracing agile methodologies, and prioritizing ethics. As we move forward, and AI integrates into our world, stay on top of the trends so you can be an AI wizard with the best of them.
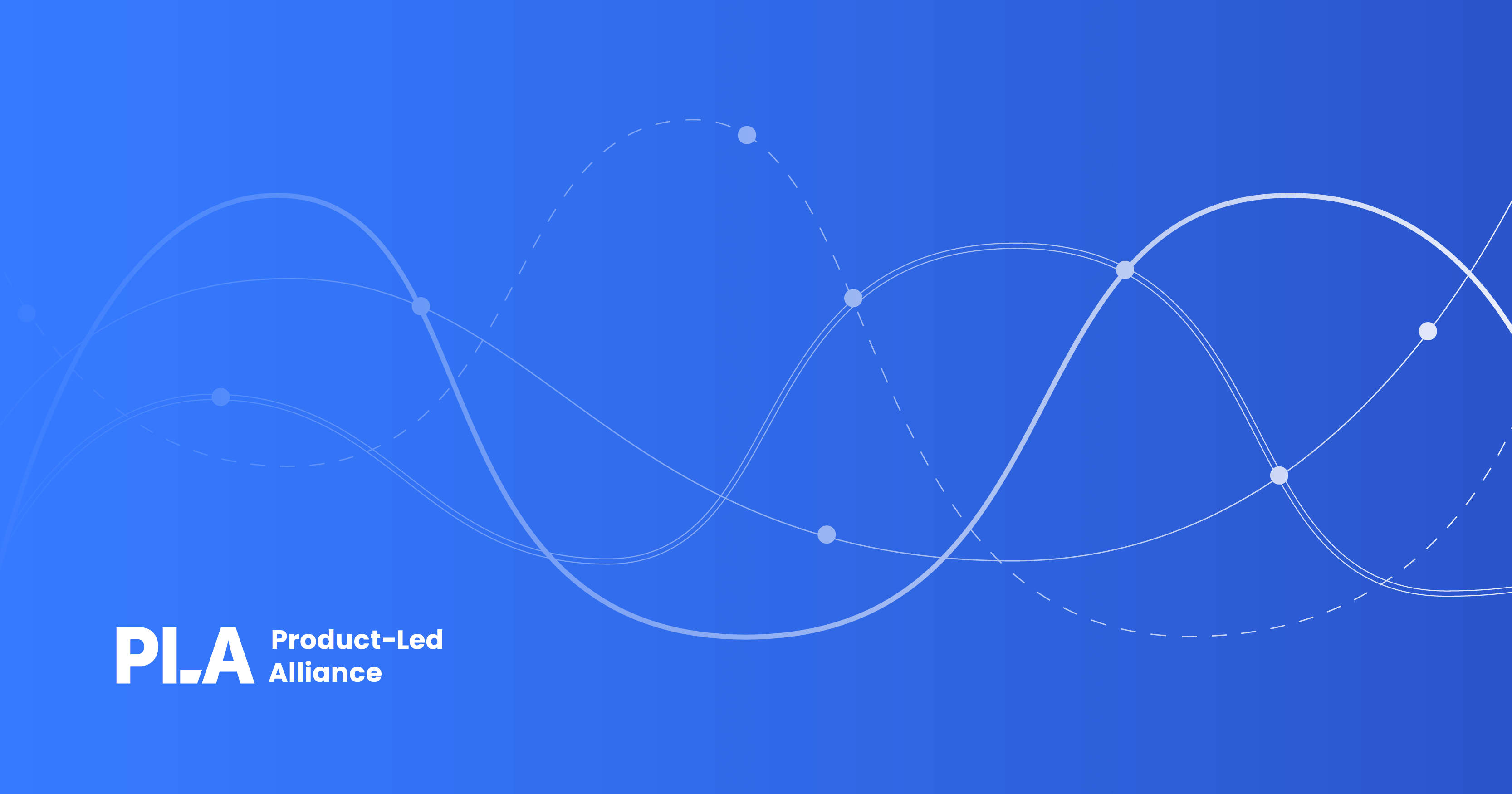
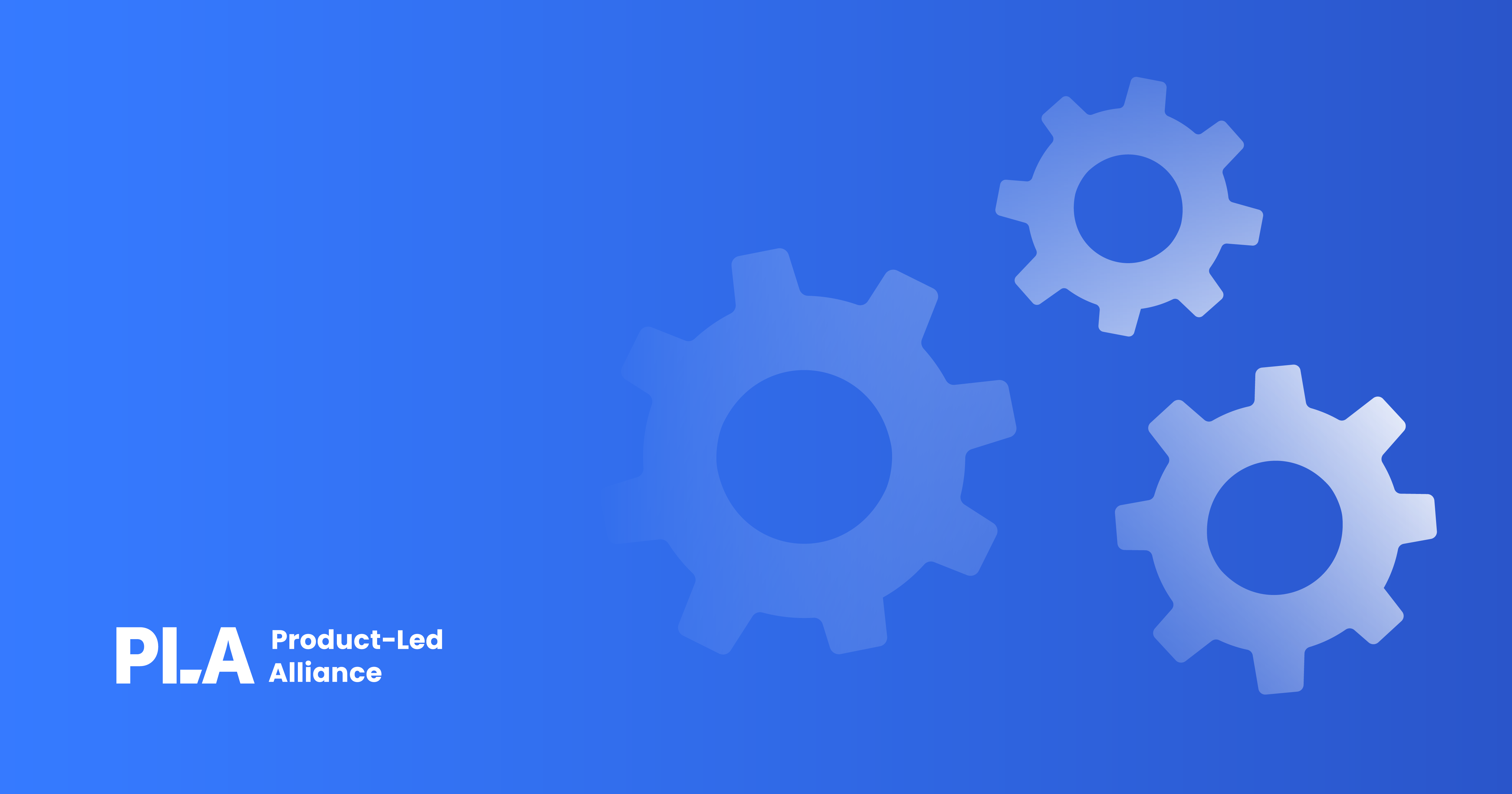
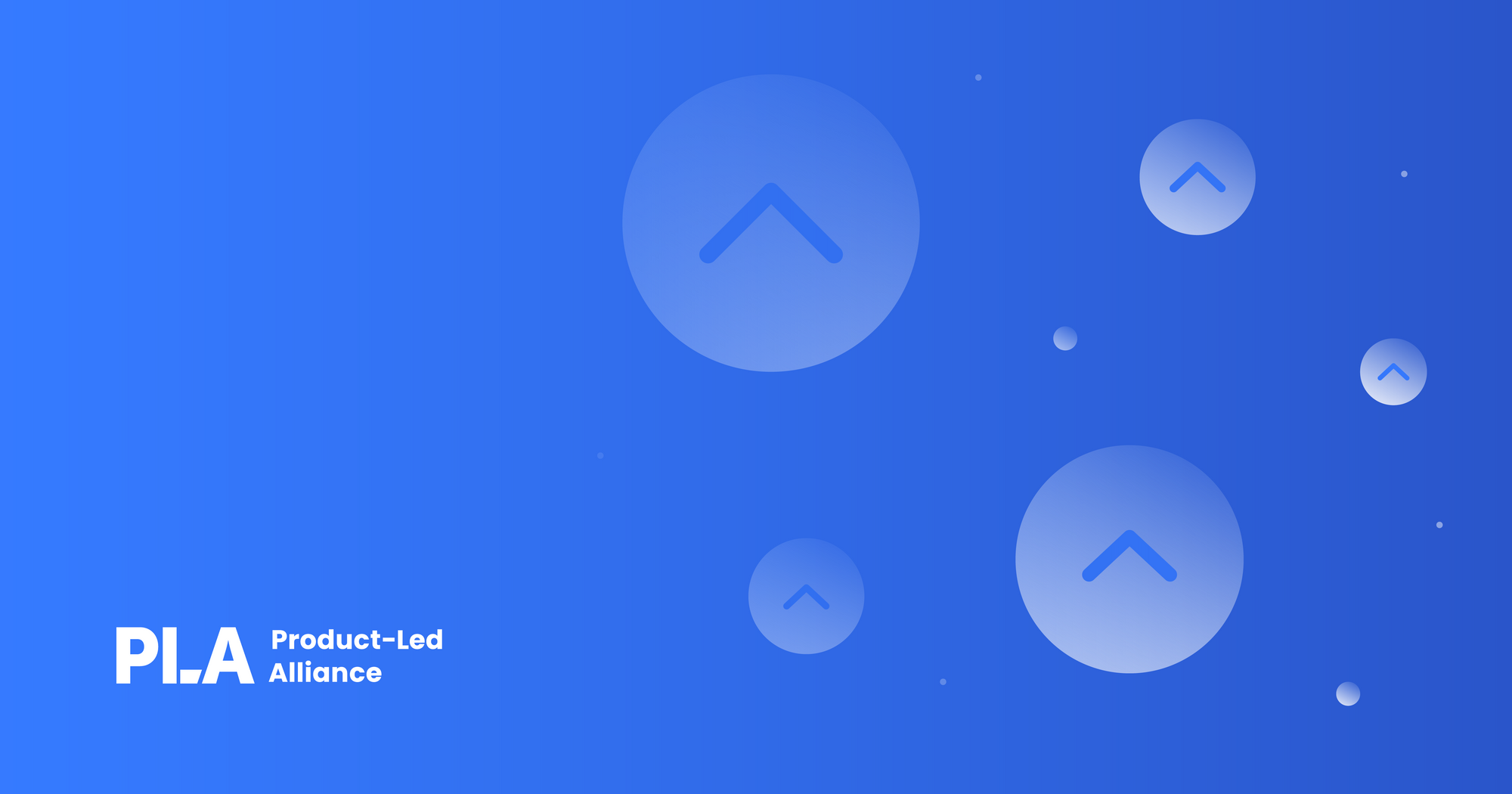
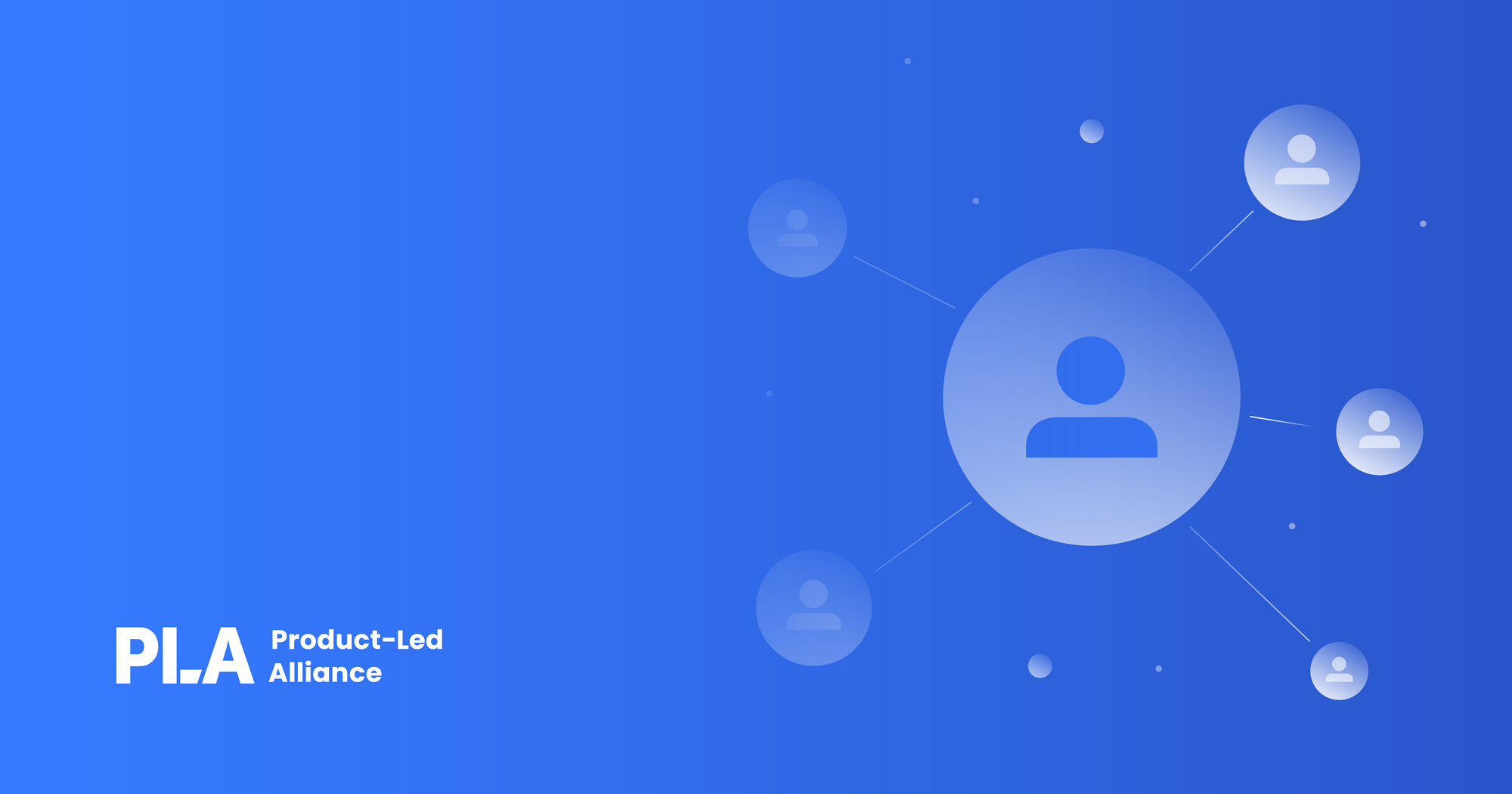