Artificial intelligence (AI) is reshaping product management. AI is no longer a futuristic concept; it’s here, woven into everything from recommendation algorithms to chatbots. It’s hard to escape it. While these innovations unlock opportunities for businesses, they also bring a host of ethical questions that product managers must grapple with. So, how do we, as product managers, ensure that we’re not only building products that delight users but also creating a positive impact on society?
Let’s look into the ethics of AI in product management, exploring key principles, real-world examples, and actionable steps to navigate this evolving field responsibly.
What does ethical AI mean?
Before diving into specifics, let’s break down what “ethical AI” means. At its core, ethical AI is about designing, developing, and deploying AI systems in ways that prioritize fairness, transparency, accountability, and the overall well-being of users. It’s not just about avoiding harm but actively striving to do good.
For product managers, this involves:
- Understanding bias: Recognizing and addressing biases in AI models that can lead to discriminatory outcomes.
- Ensuring transparency: Making it clear how AI systems make decisions.
- Prioritizing privacy: Protecting user data and ensuring it’s used responsibly.
- Fostering accountability: Creating systems that can explain their decisions and hold developers accountable.
Bias: The invisible problem in AI
Bias is one of the most talked-about ethical issues in AI, and for good reason. AI systems learn from data, and if that data contains biases—whether due to historical inequalities or flawed sampling—the system will likely replicate those biases.
Case in point: Amazon’s recruitment algorithm
Amazon’s attempt to automate hiring with AI offers a cautionary tale. The company developed an AI-powered recruitment tool that analyzed resumes to identify top candidates. However, the system was trained on ten years of resumes, most of which came from men due to the male-dominated tech industry. As a result, the algorithm began penalizing resumes that included words like “woman” or “women’s.” Amazon eventually scrapped the project.
For product managers, this example underscores the importance of scrutinizing the data that feeds into AI systems. Ask yourself:
- Is the data representative of the diverse user base we aim to serve?
- Are there systemic biases embedded in the data?
Transparency: The need for explainable AI
Imagine using a product that impacts a critical aspect of your life—like a credit scoring tool—but you have no idea how it works. This lack of transparency erodes trust, and trust is the foundation of user loyalty.
Real-world example: Apple card controversy
In 2019, Apple’s credit card, issued by Goldman Sachs, faced backlash when users reported gender discrimination in credit limits. Multiple users noted that women were offered significantly lower credit limits than their male counterparts, even with identical financial profiles. When questioned, Goldman Sachs stated that their algorithm determined credit limits but failed to provide a clear explanation of how it worked.
This incident highlights the importance of explainability. As product managers, we must push for AI systems that can explain their decisions in plain language. Transparency isn’t just a nice-to-have; it’s essential for building user trust.
Privacy: Balancing innovation and user rights
AI thrives on data, but collecting and using data responsibly is a significant ethical challenge. Users increasingly demand privacy, yet many AI systems require vast amounts of personal information to function effectively.
Striking the right balance
Take facial recognition technology, for instance. On one hand, it offers convenience and security (think unlocking your phone with your face). On the other hand, it raises serious privacy concerns.
In 2020, Clearview AI faced criticism for scraping billions of images from social media to build a facial recognition database, often without users’ consent. Law enforcement agencies used the tool, sparking debates about surveillance and the right to privacy.
For product managers, the takeaway is clear: consent matters. Always prioritize transparency about how data is collected, stored, and used. Additionally, consider whether the data you’re collecting is essential or merely “nice to have.”
Accountability: Who takes responsibility?
When an AI system goes awry, who is accountable? The developers? The company? The product manager? Accountability in AI is a murky area, but it’s crucial to establish clear ownership.
The Boeing 737 Max crisis
While not strictly an AI case, the Boeing 737 Max crisis offers valuable lessons about accountability in automated systems. The aircraft’s Maneuvering Characteristics Augmentation System (MCAS) was designed to prevent stalls but malfunctioned in two fatal crashes. Investigations revealed that the system’s design flaws weren’t adequately addressed, and pilots weren’t sufficiently trained on how to override it.
In the AI realm, product managers should champion rigorous testing and user training to ensure systems behave as intended. Accountability isn’t just about taking the blame when things go wrong; it’s about proactive measures to prevent harm in the first place.
Actionable steps for ethical AI in product management
- Conduct ethical audits: Regularly assess your AI systems for biases, transparency, and privacy risks. Collaborate with data scientists to understand potential pitfalls.
- Diversify your team: A diverse team brings varied perspectives, reducing the likelihood of blind spots in your product design.
- Engage users: Include users in the development process, especially those from marginalized groups who might be disproportionately affected by AI systems.
- Stay informed: The ethical landscape of AI is constantly evolving. Keep up with industry guidelines, like those from the IEEE or the European Commission’s Ethics Guidelines for Trustworthy AI.
- Advocate for regulation: Support policies that promote ethical AI practices. While regulation might seem restrictive, it can provide a level playing field and ensure public trust.
A glimpse into the future
Autonomous systems, generative AI, and predictive analytics will open doors to innovations we can’t yet imagine. But with great power comes great responsibility.
By prioritizing ethical principles, product managers can lead the charge in creating AI-driven products that not only solve problems but also uphold the values of fairness, transparency, and accountability. After all, the true measure of success isn’t just what we build, but how we build it.
So, let’s take a moment to reflect: Are we ready to embrace the challenge? The future of AI—and its ethical impact—is in our hands.
Looking to get your hands on the current state of data in product management? Download the report now to stay on top of all those numbers.
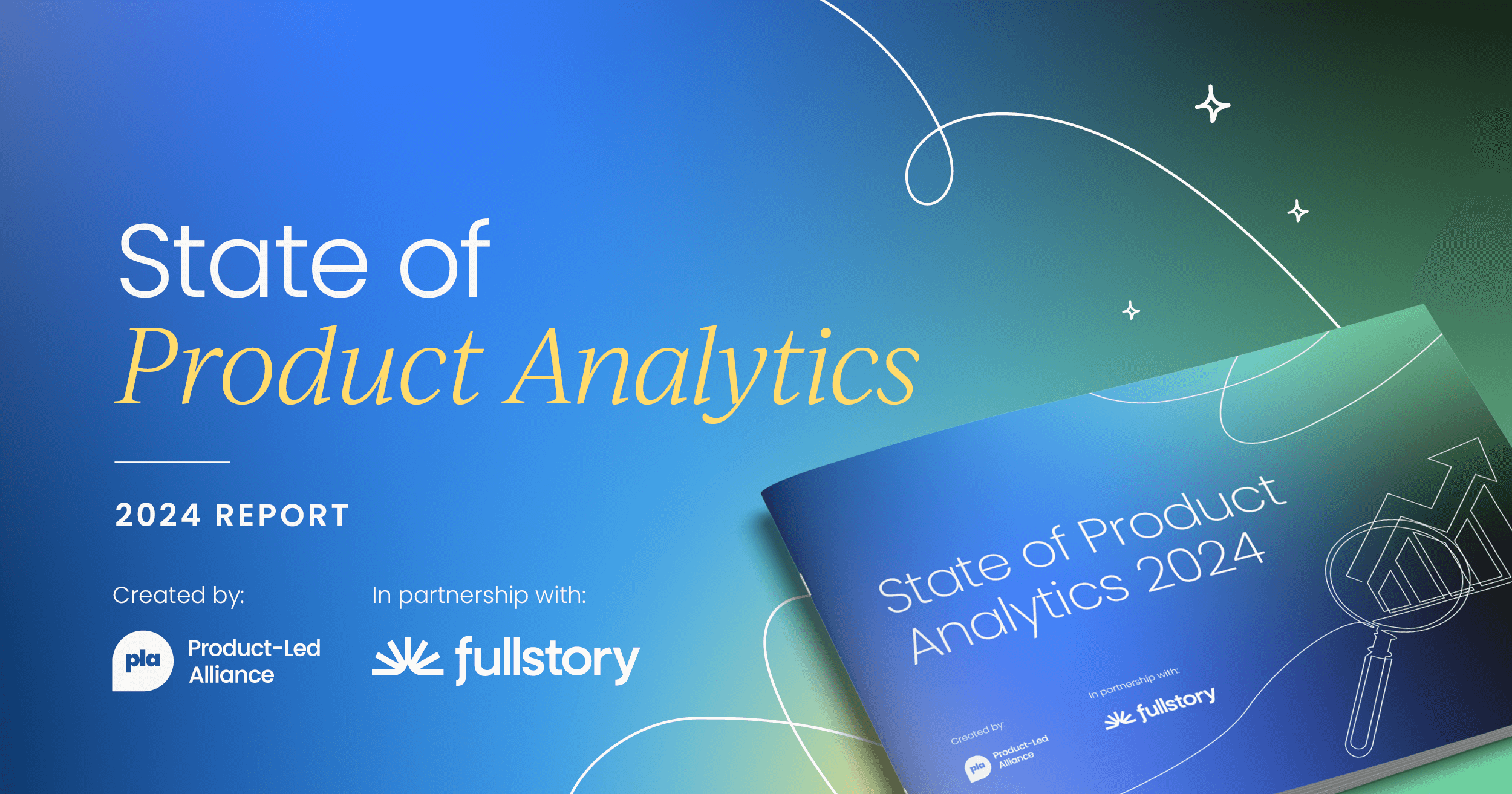