My name is Charles Caldwell, and I lead product at Logi Analytics.
Throughout my career, I focused on enabling humans to make more effective decisions by delivering data driven insight.
This article is entirely focused on AI, and rightfully so.
AI is referred to as the fourth industrial revolution, and as everything became digital in the third industrial revolution, the pace of change is no longer constrained by the linear analogue world and is increasingly moving at exponential speed.
Gartner is forecasting that in 2021, artificial intelligence will generate around $2.9 trillion of business value, and it’ll do this by saving 6.2 billion hours of worker productivity globally.
AI is already demonstrating that it can help us solve more complex problems at a larger scale, in many cases, with greater accuracy. Through applications such as supply chain, cancer diagnosis, drug discovery, and pharmaceuticals, AI is proving it can be transformative.
In this article, I'll be covering:
Challenges
Now, all revolutions have their challenges as well as their naysayers.
And in the case of AI, we can already see many of the issues we're going to have to overcome to reap the benefits that Gartner talks about without significant downside impacts. It's already clear that we're going to see job losses due to further automation, as we have with the other industrial revolutions.
In the case of AI, that automation is increasingly moving into what we would call knowledge work or so called white-collar jobs.
But we're also seeing some pretty interesting new ways AI automation is developing that we didn't anticipate, like deep fakes, bots driving our public opinion and impacting elections, or market volatility being driven by automated trading bots.
Today's narrow AI largely functions by consuming data based on past human decisions and scales that up. This can cause amplification of existing biases and all sorts of interesting, unintentional ethical issues, like the continuation of redlining and lending, bias sentencing when we use algorithms to do criminal sentencing and in courts.
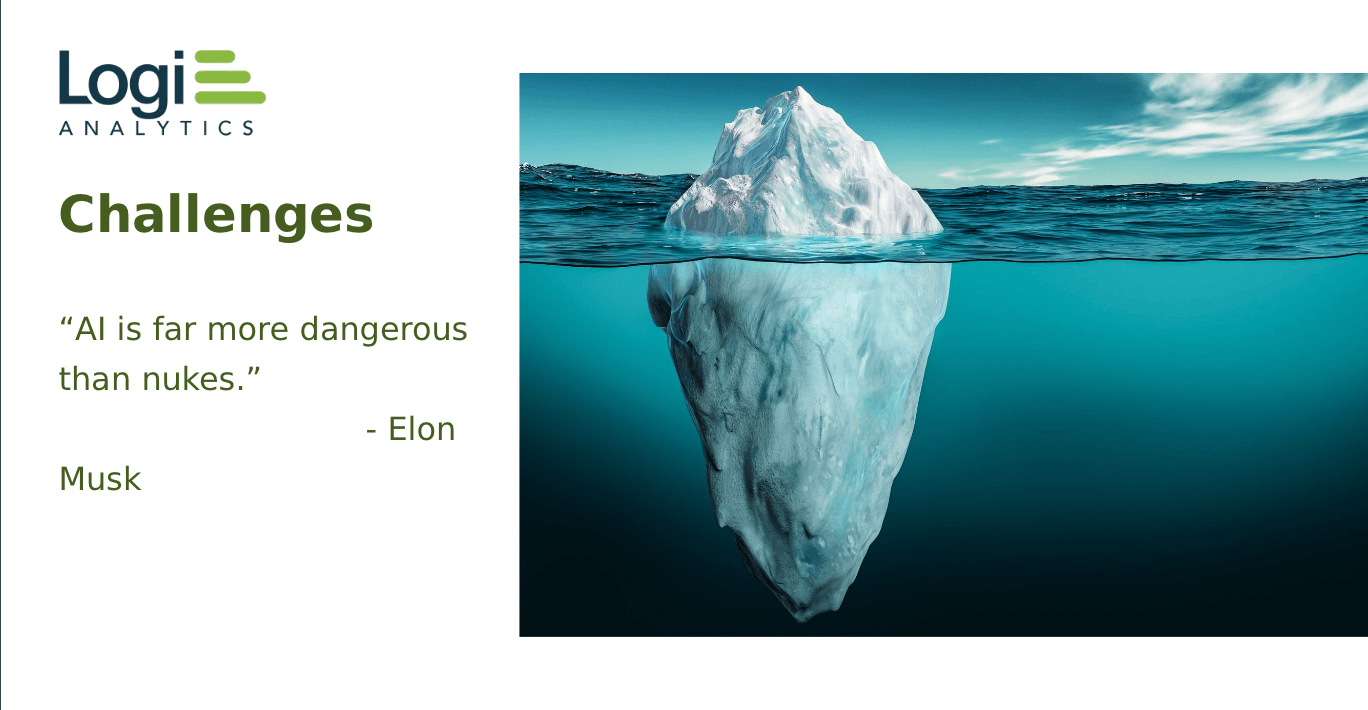
Several famous personalities have been misidentified as suspected criminals through facial recognition programs. Now, there's also these robot dogs that look super cool in the demo, but as the citizens of New York found out recently, once you put them into police jobs, the implications are a little creepy.
Maybe we're not quite ready for AI to pick up some of those policing and military jobs just yet.
AI application
Now, the reality is we're going to run this experiment. In fact, we’re running the AI experiment right now. Despite Elon Musk’s concerns that AI is more dangerous than nukes, we are applying AI to everything, including military and policing applications.
So, who's going to figure out how to gain the benefits and realize that $2.9 trillion in value while overcoming some of these very real concerns?
Now, the fact of the matter is, it's you.
Ultimately, the question of how well our AI balances these concerns will come down to the applications each of you create.
If done right, AI has the potential not only to generate monetary value but to also create a better quality of life. The first industrial revolution was totally disruptive and had lots of unintended consequences.
The Pea Soup Fog of the Industrial Revolution in London was an ecological nightmare that we had to learn to overcome. But I can't imagine anyone arguing that we should return to a time in which even the simplest projects required back-breaking human labor to accomplish.
Just as we harnessed steam and water and electricity to free us from our physical limitations, we have a very interesting and real opportunity to utilize AI to free us from our cognitive limitations.
It really is each of you that are shaping the landscape every day, in the applications you're building, and in the ways you're utilizing AI to create value in those applications.
I want to share with you just one point of view, from my perspective, on some basic things we can do, even in the spirit of trying to get this right. And it really comes out of my 20 years of experience in helping human beings benefit from analytic systems we've built in the past.
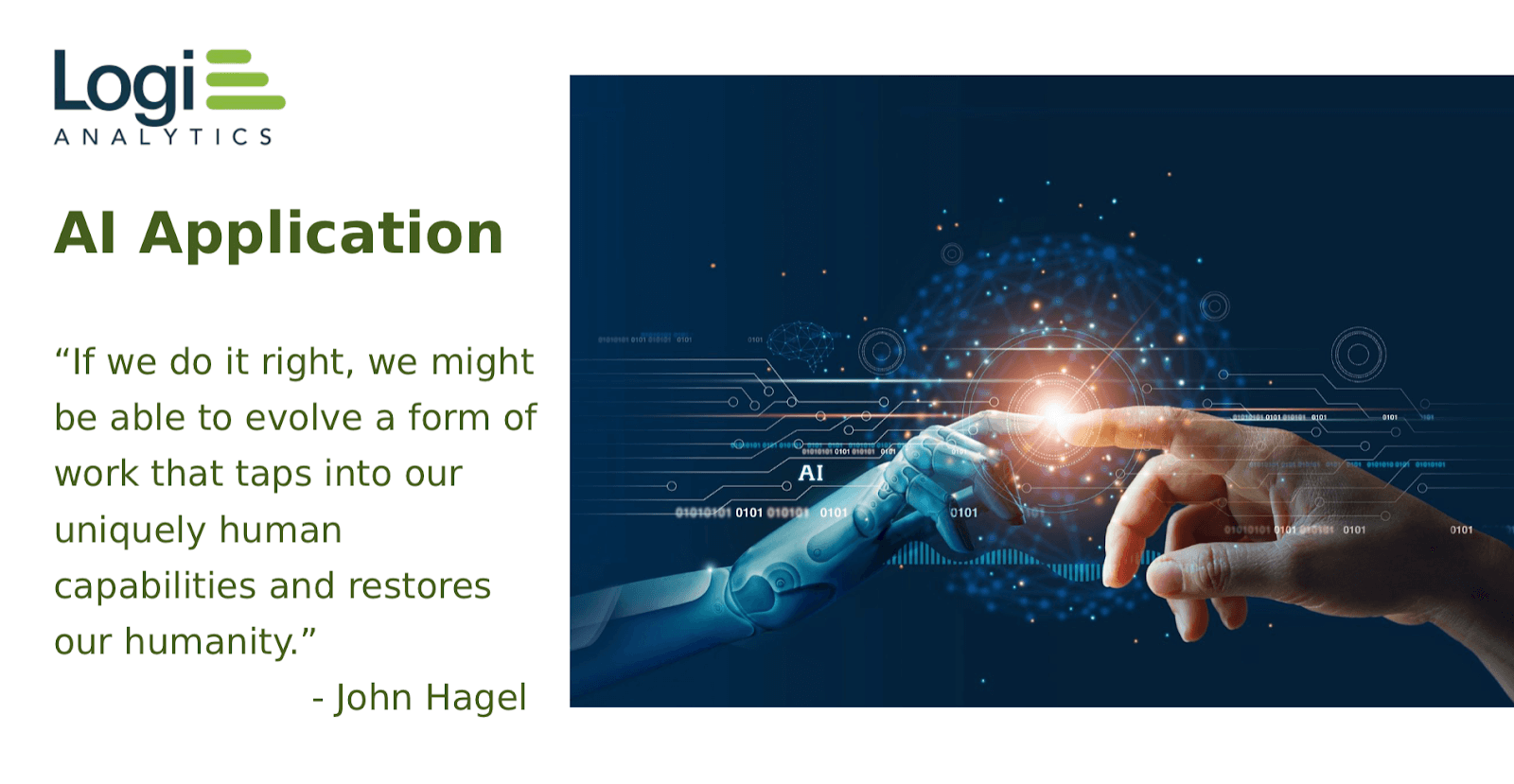
Augmenting intelligence
You can really view AI as another analytic system that we're building to help empower us humans. It really does come out of the spirit of trying to solve what exactly AI is good at and what exactly humans are good at. And then taking advantage of the combination of those two things in this fourth industrial revolution.
First, I'll start by focusing on what it looks like, what AI is currently good at, and how do we use today's narrow AI capabilities to handle tasks that humans do poorly or inconsistently? Or, frankly, just don't add that much value that we can automate through task automation.
Second, because we are still living with narrow AI, and the AI hasn't gained consciousness just yet. We need to enable humans to gain insights into how the AI is functioning, and how it's performing. And we need to provide guidance to help tune that AI and its effectiveness and gain insights.
And then finally, I'm going to suggest to you that, at least for today, humans need to stay in the driver's seat on the decision making and the action-taking. There's a balance here, of course.
So, how do we figure out where we're going to have the AI actually do the full automation of the decision? How do the end-users of our applications step in and augment those, to make sure they're effective decisions that balance a lot of these other concerns that AI currently is not able to account for?
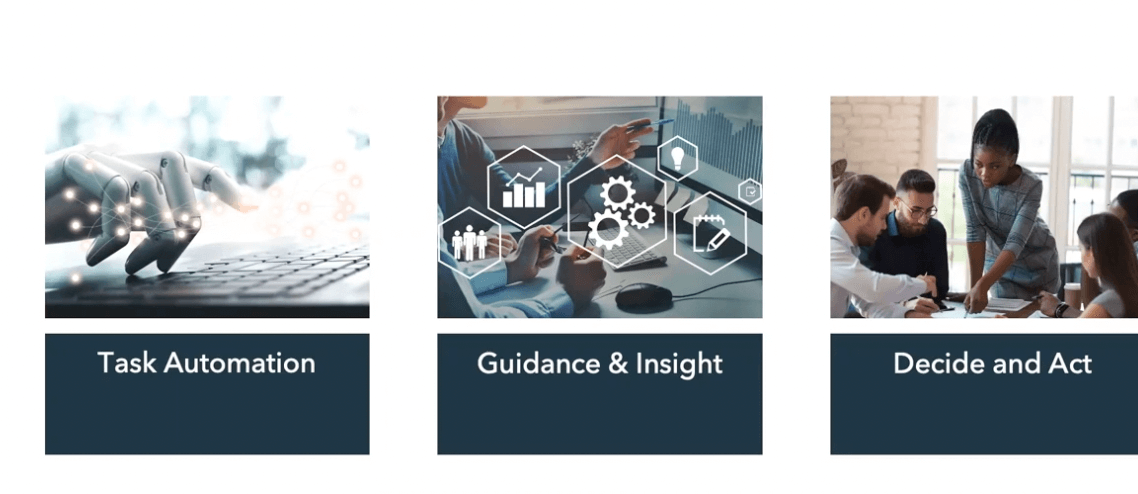
Task automation
What is AI good at?
It's very tempting to hear task automation and think about simple stuff. I can write you a four-loop with some basic steps and I'm just going to automate it, but that's not exactly what we're talking about here.
The reality is narrow AI, today, is able to do some pretty interesting cognitive tasks, such as transcribing recordings into text. Today, AI could work through that text, extract concepts from it and produce a summary that’s not just a summarization of its words, but of its ideas.
Massive data volumes
Now, not all of these have reached broad-scale application yet, and some of them can still be hard to tune. But they're really interesting examples of how narrow AI is moving into relatively complex tasks: screening for cancer, detecting epidemiological outbreaks, finding patterns of fraud...
Anybody who's been to a website recently to inquire about buying something, or to get help, has probably encountered a chatbot who's handling standard customer inquiries via chat. These are all tasks that we can solve today with narrow AI applications.
So, what are the things to look for as you think about where you can use AI to automate tasks that end users are not going to be good at?
There are a few characteristics at a high level to keep an eye out for. One of them is tasks that require processing very large data volumes because we humans tend to work from summary down.
Pattern recognition
There are many problems that you can only really solve from the bottom up, from details up.
If you look at things like fraud detection, as an example, in order for a human to look over massive data volumes to discover fraud, we're in a discovery process, slicing and dicing and looking across different groupings.
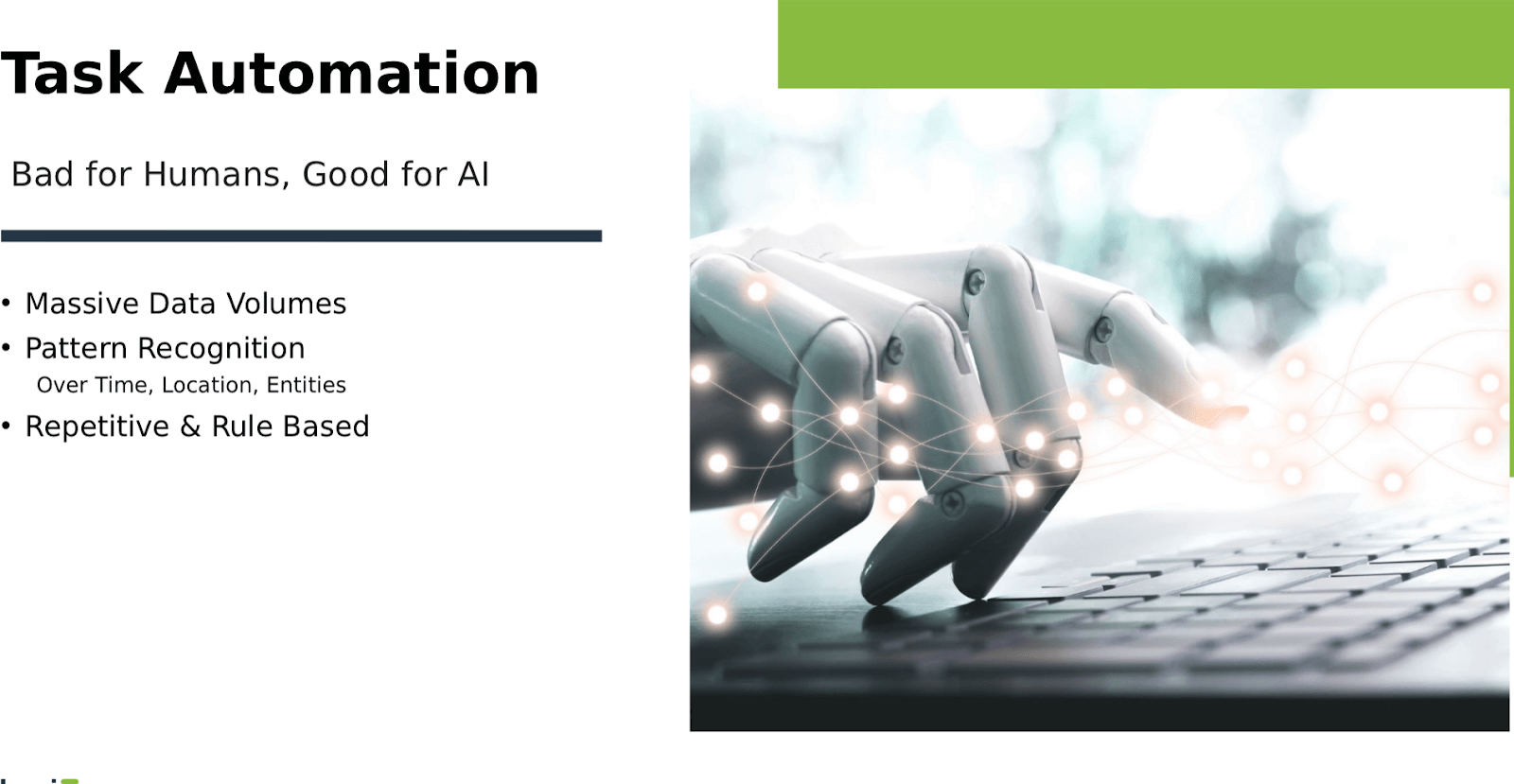
It's much more effective when we can apply algorithms from the bottom up. These types of pattern recognition are another area where the bottom-up approach is more effective and can help support human beings in ways that we're not good at.
We often get tricked when the points of data we need to correlate to detect the pattern, are spread out over time. That's one that will sort of trick a standard human discovery process. Or they're spread out over location or any other grouping entities in the data.
And again, this is where the bottom-up approach from an AI algorithm standpoint works, because those algorithms can look at what does correlate to specific outlier patterns and specific metrics, to discover the relationships where human beings tend to come hierarchically into the data, starting with relationships to try to find the patterns.
Repetition
The third one is really just repetition. And especially if I can apply algorithms and rules to solving highly repetitive tasks, that's an area where maybe we humans are okay at.
But we can be inconsistent?
We don't scale as quickly as the robots do, and this is why we're seeing chatbots everywhere.
So, if I've got 10,000 customer inquiries all coming in at once, and scaling up a team of human beings to solve for all of those. The vast majority of them are going to be things like:
“What is your current mortgage rate?”
Or
“How do I open a checking account?”
And it's just not high value to have a human being fielding that. So, we get a chatbot to field that answer. That's just about very repetitive tasks, and repetitive also means at high scale.
So, if I'm getting the same question all at once at a high scale, these are all areas that are really good candidates for exploring where AI can help relieve humans of these tasks. To free them up for higher-value engagement in the process.
Guidance and insight
The next thing you want to think about in user experience is how to engage humans to help provide guidance, insight and make up for any gaps in the AI where it's not going to perform well.
One of the things that we have to deal with almost immediately with AI is the fact that a lot of AI algorithms are not explanatory in nature. So, with an explanatory model, its goal is to tell you why you arrived at this prediction, what seems to matter?
What correlates to a record being fraudulent so we can start to understand what causes fraud?
All models are black boxes
Most AI techniques that get used for these things cannot tell you why: they're not built to tell you why, they sort of give you a decision that gives you an outcome. And they're not able to explain exactly what mattered in producing that outcome.
But frankly, even when they can at scale, you really want human beings dealing with monitoring the process for effectiveness and scale.
So, look at:
- What is being recommended?
- Are those recommendations correlating well to the outcomes that we're trying to produce?
- Are those outcomes getting better? Or worse?
And you also want to help detect unintended consequences:
Is our AI automation unfair or biased in some way? Are we accidentally breaking some laws because of the way the AI is causing it to do something in violation of a regulation? We need to be able to detect those things and then provide guidance back into the system. And this is really a balance about, again, knowing what the AI is good at.
Provide insight into AI “decisions” and “outcomes”
AI may be really good at detecting potential fraud. But then you want a human being to intervene and decide if this is really a fraud or not. You want them to be able to understand what the AI is detecting to potentially give them some information about why the AI is saying that.
Then have them provide guidance back in and I'm sure all of you have experienced this at some point when you get an email or a text from your bank saying, “Hey, I’m not sure this transaction is you..”
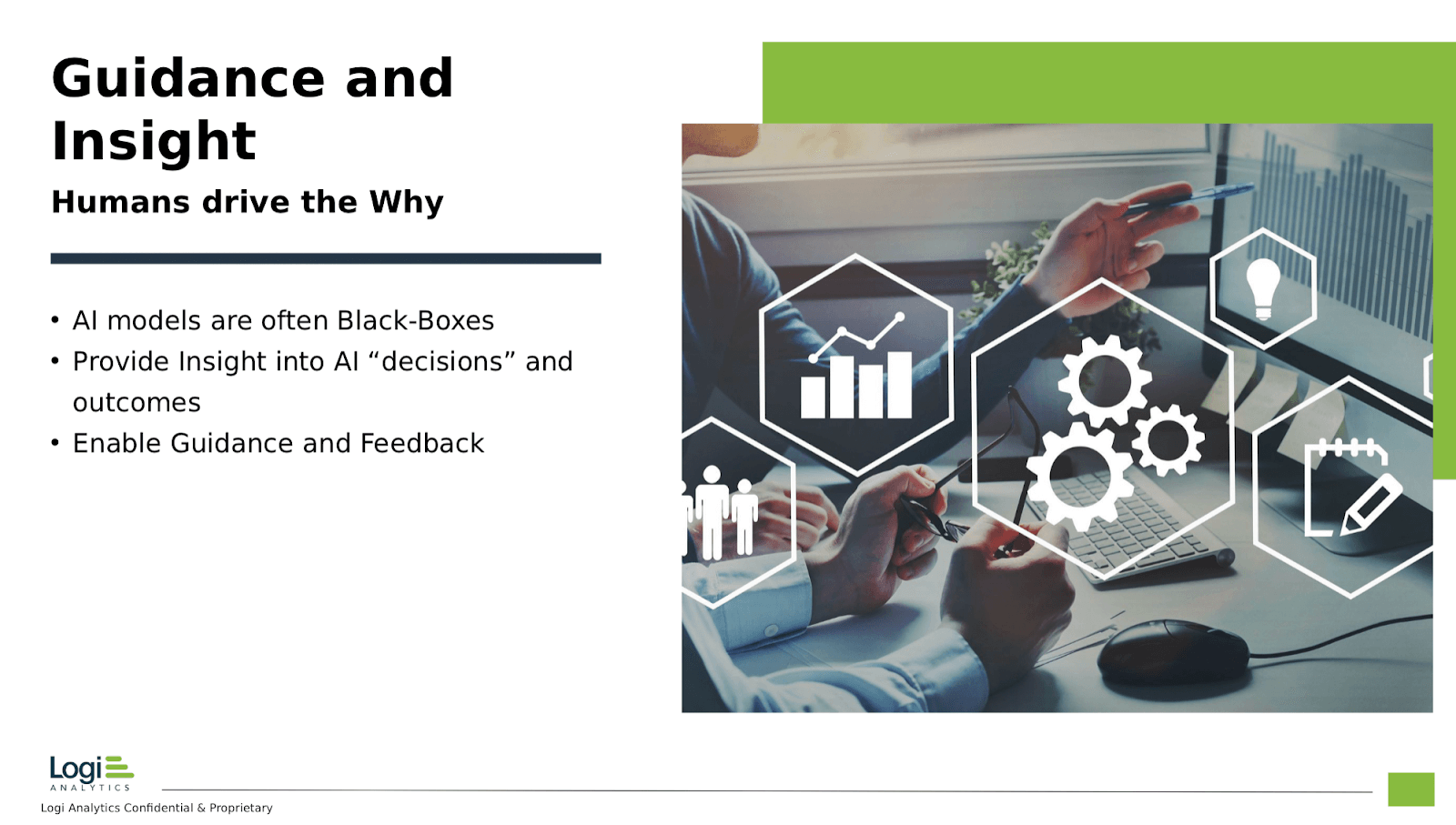
So, in the flow of your application where you're using AI to do the task automation, you want to find opportunities for engaging human beings, where appropriate, to get insight into how effective that process has been and to provide guidance.
Was this a good recommendation?
Is this a fraudulent transaction or not?
Then, if you’re using the AI correctly, it will also learn based on that feedback and guidance, while improving over time as well.
Decide and act
Some folks will disagree with this opinion, but certainly, for now, I think humans need to call the shots. So, we do need to be very careful when considering how much of a decision we want to completely outsource to AI, and where we want to call in the judgment of a human being to make the call.
Is the decision appropriate for AI?
One of the things to understand here is: How do you tune AI for decision-making?
Basically, as you're tuning your AI, you’re either skewing it to find every possible instance of concern, which means it's going to convict some innocent people in that metaphor, and it's going to find every possible instance and get a lot of false positives. Or it's only going to identify the real instances.
In which case, the guilty go free. You’re going to miss some stuff.
And the reality is you can build AI systems that will balance this. So, part of it will find every possible one, the next part of it will try to focus on only the ones that absolutely meet the criteria.
At some point, though, you sort of run out of the ability to optimize that.
For a variety of reasons, it could purely just be down to the capabilities of the AI, but it can also be about any moral, ethical, and legal concerns you want to hand off to a human being. An example that I use here is loan approvals.
I've seen lots of AI approve loans, I don't see many AI rejecting loans. And yes, those are two different things.
So you can go onto a website, fill out some forms. And if it's clear, according to the algorithm, the rules that have been established, that you're a good loan risk, it will be approved. But if it's not clear, they don't let the bots reject you, they will push that decision up to a human being who will dig deeper. This is still an analytic process.
I'm not saying we suddenly go off into a non-data-driven process. But you're now using the more generalized intelligence of a human being to be able to handle some of these other concerns.
For any of you who have heard me speak before on the topic of embedded analytics, and how to deliver insights in the context of an application, you’ll really always hear the same three recommendations from me.
Deliver AI insights to human beings
When you're bringing these types of insights to human beings, you want to make sure they're in context for that person, that the insights are structured in the same way the decision is structured.
What’s important to the decision is how you're presenting the information. And then as much as possible, all of this is as close to the action-taking step as it can be. So once the decision is made, the person is pressing the button that kicks off the action resulting from the decision.
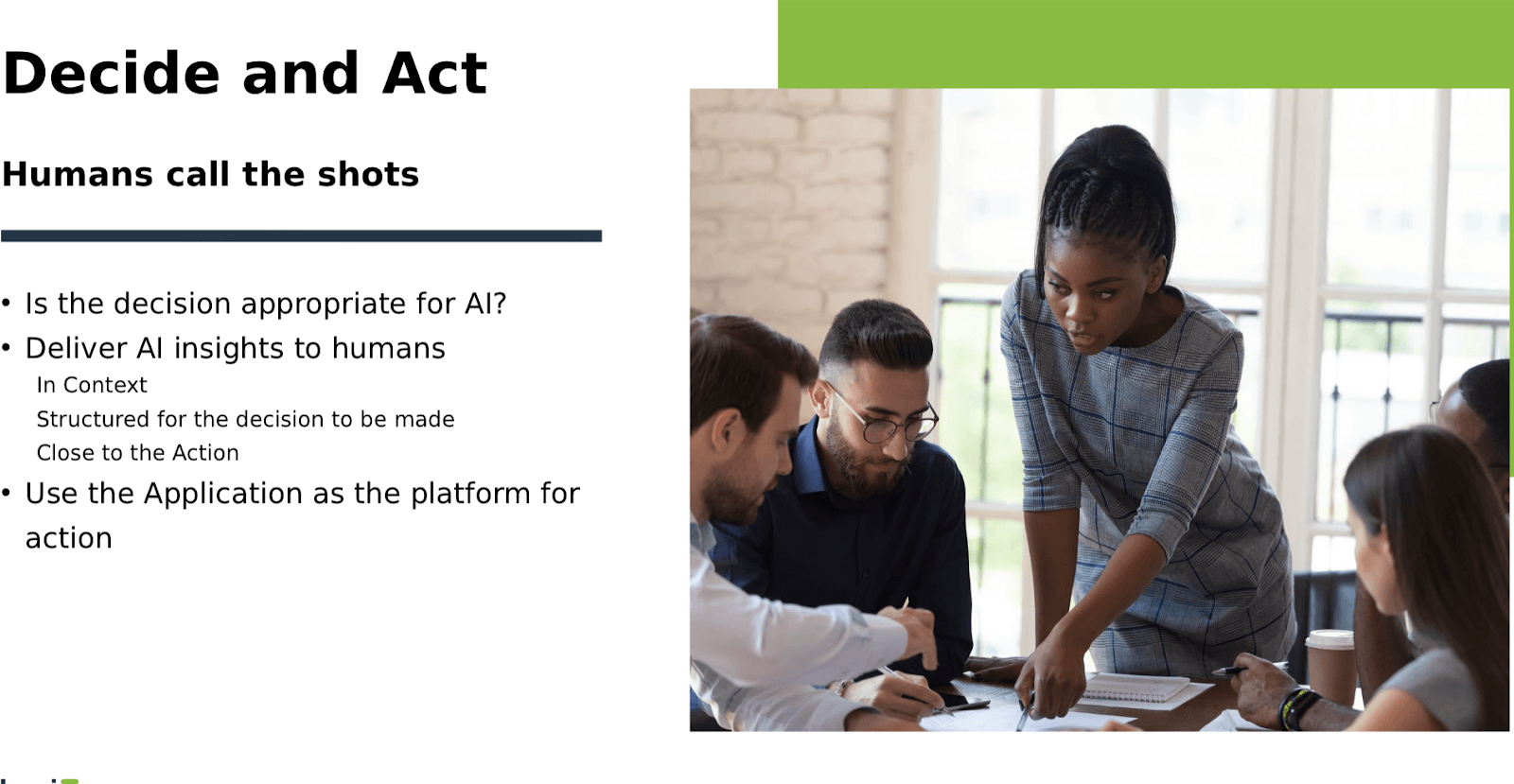
Use the application as a platform for action
You want to get at this gap between knowing and doing now, like in the context of a loan application. You want to make sure that person has context by having them understand - here’s the full view of the loan for review, and here's why it was not approved.
Before you saw it, whether that was the bots that couldn't approve it, or another person who couldn't approve it for some reason. What's anomalous or different about this application that we want you to review?
Yes, I want related information, but give me the information that matters the most so it’s structured for the decision-making process. So, we need you to look at collateral income, credit score, whatever it is that's outside of the typical boundary for approval.
And then, having completed my analysis, give me what I need to move this to the next step, whatever that is.
Maybe I need to change the amount, the terms, the rate, and approve the loan. Give me the ability to do that right there. Don't make me toggle between systems. Keep it all together in this main app.
As much as you're following that structure for enabling that humans get this handoff from the AI, you need to understand:
- What decision is being made?
- The information needed to make it.
- The ability to get that decision going right there in the app.
Your application does become a very powerful platform for your users to gain the benefit of AI. As well as gain the insights, and then, where the AI is not able to carry the task automation all the way through decision and action, to add real value.
They’re adding their best value. It's non-repetitive, it's discretionary. And they're helping to overcome the concerns that the AI frankly cannot. Ultimately, the promise of AI is not about replacing humans, of course, but it’s about providing augmentation to our intelligence to make us more effective.
Much like the first industrial revolution was about doing heavy lifting in the physical world, AI very much is about helping us do more heavy lifting beyond our natural capabilities in the cognitive world. Helping us make more complex decisions, make them at a higher scale, and make them more effectively.
Realizing that is the foundation of all the applications you are all building right now. And those applications can help remove the burden of those lower-value cognitive tasks by utilizing AI. They can enable your end-users to gain insights based on how the AI is performing and provide guidance back into those decisions to improve the UI over time.
Finally, they can leverage those insights to enable your end-users to really focus on the high-value decisions they need to make and then facilitate the action. That is the result of that decision
In using that combination of approaches, your applications can deliver massive value to your customers. Frankly, if you get that balance right, you’re going to help address Elon Musk's concern that we may run off the rails by just scaling up out-of-control bias decision-making.
We are balancing all of these other concerns that we as humans are much better at dealing with today than AI.